Can Smoking Cause Breast Cancer?
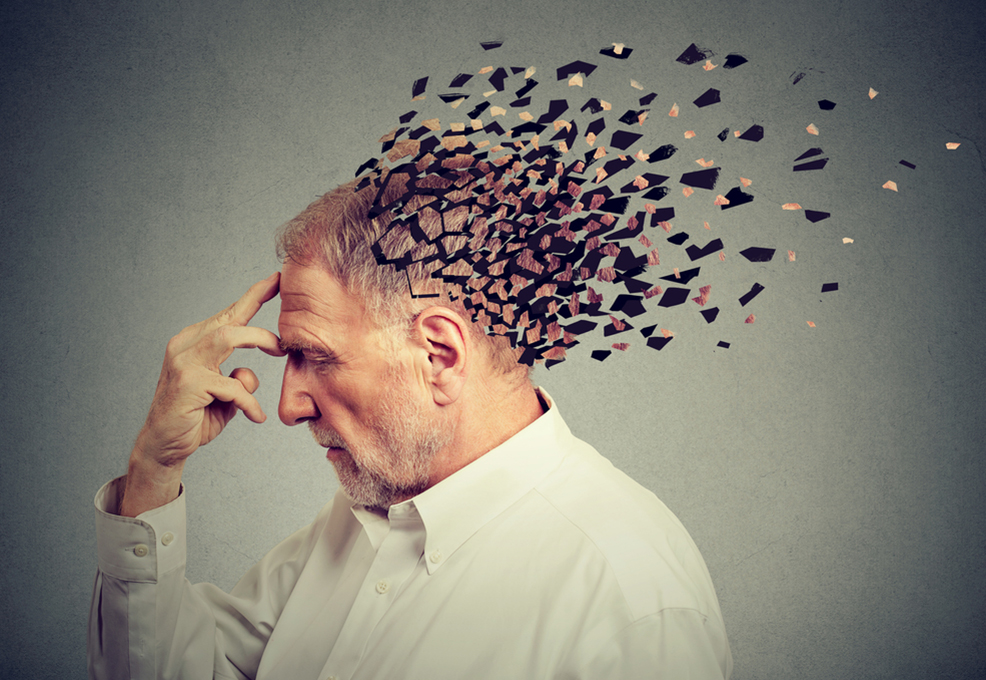
Author(s)
Shu-Lan HsiehBiography
Prof. Hsieh is currently a distinguished prof. at the Department of Psychology of National Cheng Kung University (NCKU). She is also jointly appointed to the Institute of Allied Health Sciences and the Institute of Public Health at NCKU.
Academy/University/Organization
National Cheng Kung University-
TAGS
-
Share this article
You are free to share this article under the Attribution 4.0 International license
- LIFE SCIENCES
- Text & Image
- January 22,2019
A healthy brain is essential for enjoying a productive human life. As people age, brain function deteriorates and manifests its declining performance at the cognitive level, thus increasing the economic burden of aging. This burden has become a global health issue. Yet, since people age at different rates (including brain and cognitive function), developing a fast and non-invasive method for regular monitoring of an individual’s brain health status would help us undertake early detection and identification of those at risk of age-related cognitive decline or illness problems years before the symptoms appear. For future prospects, once we identify the early signs of problems based on individual predictions, we can provide a client-based up-training intervention program to ameliorate these early symptoms and boost an individual’s well-being. Our technique so far can accurately predict an individual's age and cognitive performance based on fast resting-state acquisition and machine learning algorithm. Our current predictor has the second highest accuracy among published MRI-based age predictors around the world.
When someone asks you if you know how old you are, you might think that this question is stupid. Suppose someone asks you if you know how intelligent you are or if you know your test score, you would probably say “yes, of course I know”. Your answer is simply based on your prior knowledge about your IQ score and other test scores. Yet, sometimes these take-for-granted questions can become very indeterminate and difficult to evaluate. Although you can easily estimate your own chronological age based on your birth date (let’s say 65 years), your brain or mind’s age may not be exactly 65 but may actually be much younger or older than that. That phenomenon occurs because aging does not affect people uniformly. Many factors, including environment, social network, occupation, and lifestyle may influence the brain’s health. Therefore, some individuals’ brain may decay faster than others, while others may be preserved better than their contemporaries’ brains. Although there are numerous psychometric tests and examinations available to assess an individual’s intellectual ability with regard to cognitive competence, some individuals may have difficulties in completing pen-and-pencil tests or following the tests’ instructions. Therefore, we need to develop a technique in which an individual’s ability can be predicted through his/her brain activity without having to perform any task. Yet, sometimes, even when an individual is healthy and capable of answering the evaluation instruments, she/he may not have sufficient time to undergo a thorough evaluation for an extensive number of hours. Furthermore, if we want to regularly check an individual’s brain and cognition health status in this scenario, there will be a profound confounding practicing effect even when an individual has plenty of time to take repeated evaluation tests. Therefore, developing an objective and easily accessible evaluation method for the brain and cognition function is needed.
Understanding the fact that the above-mentioned originally taken-for-granted questions are no longer as straightforward as we thought, many large international neuroimaging projects across various nations and organizations have therefore launched extensive projects in order to tackle these issues. The idea has been to teach machine-learning algorithms that could correctly classify and discriminate between healthy and diseased brains. For example, some projects have claimed to be able to predict brain age, mortality age, mental illness risk, and/or dementia onset. Yet, although these projects already utilize artificial intelligence (AI) to predict age or disease onset, they focus to a lesser extent on predicting an individual’s cognitive performance. Therefore, our research team conducted a series of experiments by combining brain imaging data and machine learning algorithms in order to identify patterns in neuroimaging data aimed at predicting individuals’ executive functions and internet addiction tendencies.
The data we analyzed consisted of resting-state functional magnetic resonance imaging (RS-fMRI) data that were collected from an MRI scanner. fMRI measures the quantity of the oxygenated hemoglobin in the blood that has been converted to the deoxygenated form. Therefore, such blood-oxygen-level-dependent (BOLD) responses are an important physiological signal because they measure the consequences of neural activity. Low-frequency fluctuations in the BOLD signal are a fundamental feature of the resting brain, and their presence is a key for determining correlated activities among different brain regions. In our studies, we sought to establish the credentials of slow fluctuations in brain activity as a biomarker of individual differences in brain-related aging processes and cognitive functions using a predictive modeling machine learning-based approach and applying it to raw resting-state fMRI data.
By using this technique, we have been able to successfully predict individuals’ multitasking control abilities, inhibitory functions, and internet addiction tendencies via eight minutes of resting-state fMRI in conjunction with a machine learning algorithm. In addition, our technique can accurately predict an individual's age, and our current predictor has the second highest accuracy among published MRI-based age predictors and the first highest accuracy among published worldwide fMRI-based age predictors. These achievements were chosen to be presented during the press conference held on July 20, 2018 that was organized by the Ministry of Science and Technology (MOST) and later were elected to be demonstrated at the
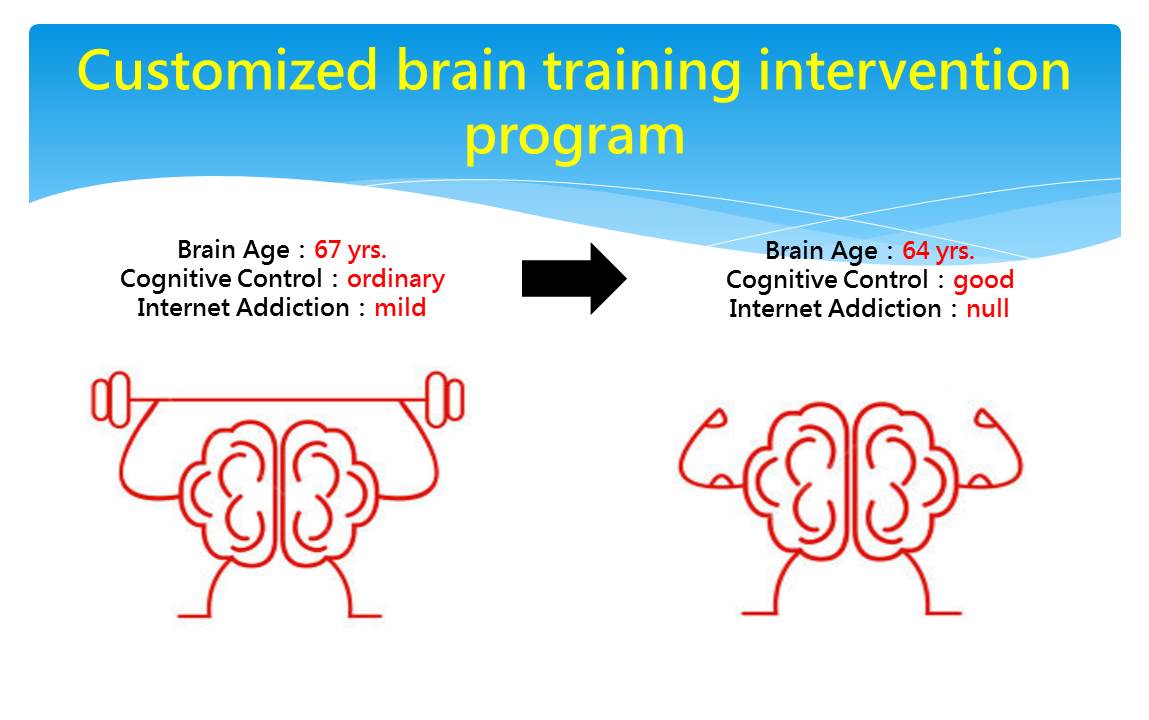
STAY CONNECTED. SUBSCRIBE TO OUR NEWSLETTER.
Add your information below to receive daily updates.