A New Era of Navigation, Starting from Space-Big Data in the Ocean
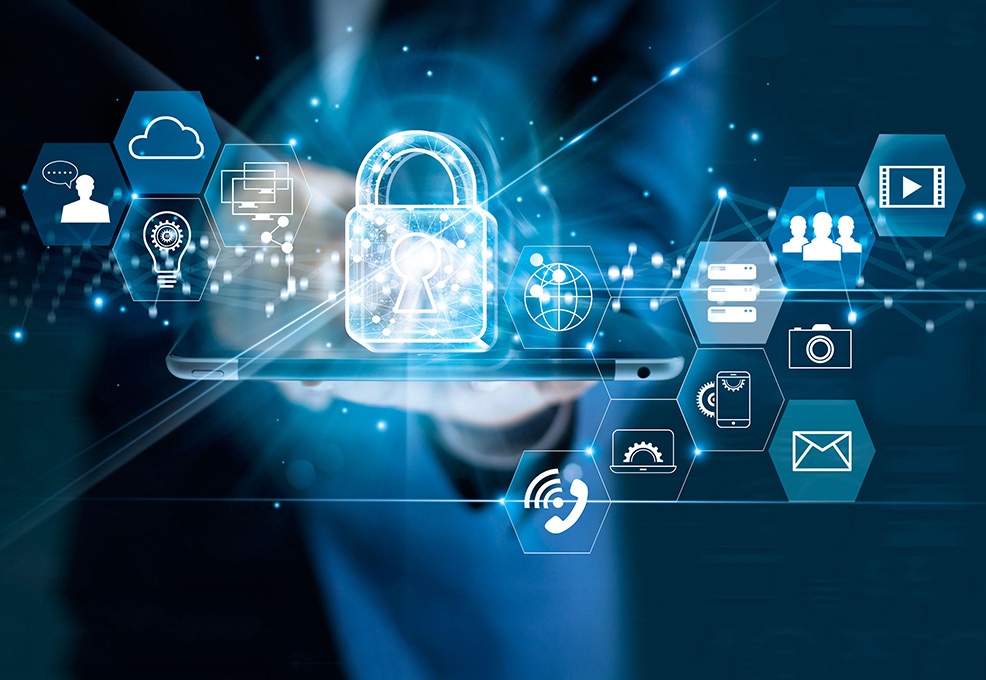
Author(s)
Wei-Chung HwangBiography
Dr. Wei-Chung Hwang, Director of the Industrial Technology Research Institute (ITRI). He specializes in Public Key Infrastructure(PKI), big data, data privacy, and AI. He is highly interested in data science and new technologies for intelligent identification.
Academy/University/Organization
Industrial Technology Research Institute-
TAGS
-
Share this article
You are free to share this article under the Attribution 4.0 International license
- ENGINEERING & TECHNOLOGIES
- Text & Image
- October 18,2021
Artificial intelligence (AI) applications have gradually matured on the Internet, the cloud, and mobile devices. Enterprises and governments that use AI technology can stimulate their efficiency or derive innovative services, helping them obtain a more generous profit. According to statistics from Statista, the global AI-related market size in 2020 was estimated to be $22.59 billion, an increase of 53.8% from the $14.69 billion in the previous year, and it is expected to grow to about $126 billion by 2025, which shows that AI applications are booming sustainably.
One critical challenge of AI applications is that the data with personal information, such as medical, business, or government data, cannot be used directly because of the regulatory requirement to protect personal privacy. Data need to be properly protected or processed for the follow-up value-added analysis and research.
To address this challenge, the R&D team of the Data Privacy and Platform Technology Department of Computational Intelligence Technology Center (CITC) in the Industrial Technology Research Institute (ITRI), has developed three de-identification methods to assist data owners in utilizing the value of data without violating personal privacy, including K-anonymity, the Generative Adversarial Network (GAN) generating synthetic data method, and the multi-agency statistical integration method derived from GAN. The basic principles and practices are explained as follows:
1. K-anonymity: This is not the most powerful method. However, it is the most intuitive and easy-to-operate traditional de-identification method. Data suppression and generalization were used, that is, data description for generalization and abstraction, for instance, an exact value is transferred to an interval for achieving anonymity. The personal data, directly identifying a natural person (such as name and ID number, etc.), are encrypted, replaced, or deleted; the indirectly identifying information (such as age, address, etc.) are hidden in a specified range. The range from 21 to 30 years old is changed to 30 years old, and the range from 11 to 20 years old is changed to 20 years old (Figure 1). Coarser data granularity increases the difficulty of data inference and attains concealment-preservation because more people will meet these interval conditions.
Figure 1. K(2)-anonymous
2. GAN generating synthetic data method: GAN is used to generate synthetic virtual data, which is similar to the real data to achieve an anonymous and analytical effect. The principle of this method is shown in Figure 2. Random variables are used as the inputs to the GAN generator, which generates synthetic data with the characteristics of the original data. Then the GAN discriminator is used to judge the fidelity of these synthetic data while it feeds back the discriminant results in each round to adjust the generator, thereby improving the authenticity of the synthesized data. This iterative process will be repeated until the synthetic data generated by the Generator successfully deceive the Discriminator. If there is a need for higher strength privacy protection, differential privacy will even be added to increase the intensity of de-identification. Compared with the original data, the analytical accuracy of the synthetic virtual data generated by GAN in all machine learning methods fell within a tolerance interval of 5%.
Figure 2. Use GAN to generate synthetic data
3. Use GAN to de-identify and re-fuse: Integrate GAN and traditional statistical methods, which can be used for data de-identification and integration analysis among multiple agencies. This method first generates data from GAN at each agency, then through the method of database join and record linkage it is possible to obtain analyzable integrated data. This integration method can be divided into 3 steps: (1) generating data with GAN at each agency, (2) integrating these data with database join, and (3) exporting the de-identification data with analyzable ability. For example, Bank A and e-commerce B have datasets X, Y, and X, Z, respectively, where X is an intersection of A and B, and Y and Z are related to X. To merge data while maintaining personal privacy, two agencies first de-identify their datasets by GAN. Then the synthetic datasets can be integrated by mechanisms such as database join, record linkage, and statistical matching, etc. Finally, the integrated datasets X, Y, Z can be used for the further analysis process.
To date, Taiwan’s government has established two national standards: CNS 29191 and 29100-2 for the de-identification and verification of personal data. Under these standards, CITC has worked with an external legal team and academia to provide the total solution and consultation service for personal information de-identification. CITC has assisted many government agencies, financial institutions, and telecom companies to leverage data and analytics in their services and business. In the future, CITCT will continue to develop more technologies for data and AI governance, to advance the innovation of AI applications for Taiwan’s government and industry.
STAY CONNECTED. SUBSCRIBE TO OUR NEWSLETTER.
Add your information below to receive daily updates.