Optimal detection of spatial modulated signals in 5G and beyond: Agorithms and hardware architectures
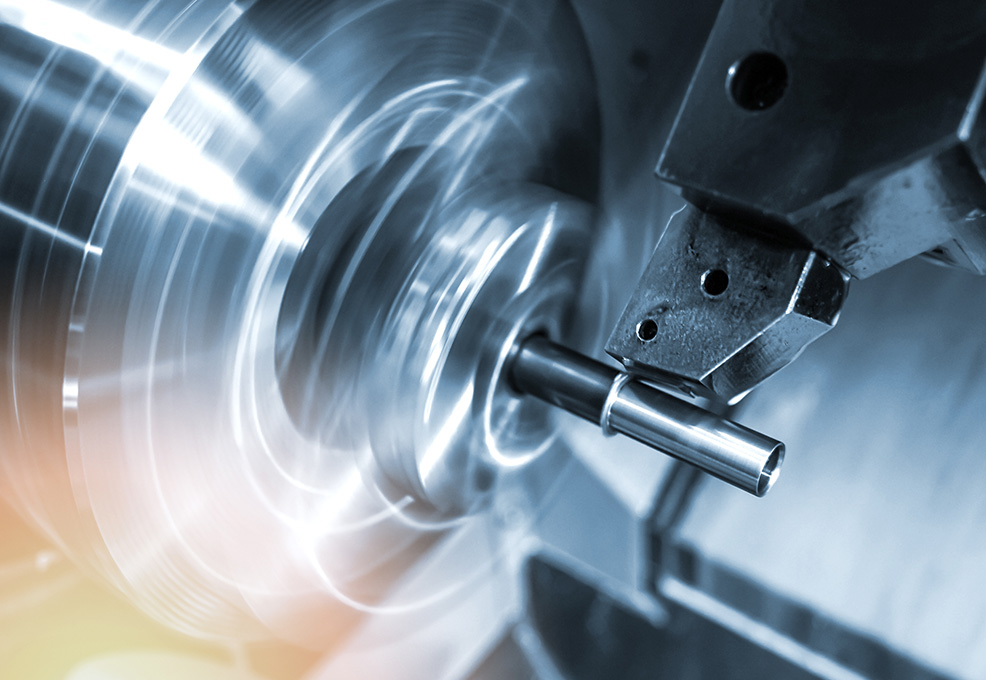
Author(s)
Yeau-Ren JengBiography
Yeau-Ren Jeng is Endowed Chair Professor of National Cheng Kung University, Founding Director of the Advanced Institute for Manufacturing with High-tech Innovations (AIM-HI) and a Professor of Biomedical Engineering. He is a renowned scholar in the areas of tribology, nano-mechanics, and characterization of mechanical properties at the nano-scale. Also, he holds over 20 patents and has received numerous awards including the McCuen Special Achievement Award from General Motors, the Innovative Research Award from the American Society of Mechanical Engineers, Society of Tribologists & Lubrication Engineers Walter Hodson Best Paper Award and Captain Alfred E. Hunt Memorial Medal, the Research Invention Award from the President of Taiwan, and the Outstanding Research Award from Taiwan’s Ministry of Science and Technology.
Academy/University/Organization
National Cheng Kung University-
TAGS
-
Share this article
You are free to share this article under the Attribution 4.0 International license
- ENGINEERING & TECHNOLOGIES
- Text & Image
- August 17,2021
The machining process requires extensive experience accumulation for proper cutting tool selection, setting of operation parameters, and timing of tool replacement. Our data-driven knowledge base of metal machining reduces the need for such a costly, time-consuming process, and improves overall logical management. With the growth of the data bank, our deep learning process can further provide the optimal operation parameters in different scenarios to meet the demands of ever-challenging business environments, increasing stringent precision requirements, and the proliferation of cutting tools and new materials.
The data bank of this knowledge base of machining includes cutting thrust monitoring with an embedded force sensor, motor current from the controller, selective cutting temperature monitoring with infrared sensors and surface roughness characterization, and cutting chip analysis in the laboratory. The in-house designed embedded force sensor not only provides an efficient, cost-effective approach for machining tool life prognosis, but further provides vital insights into a cutting process to establish the knowledge base. The accumulation of the data amount within the data bank would allow the use of our knowledge base in the basic machine tools without the embedded sensor for thrust force monitoring to provide much-needed guidelines of the timing of tool replacement.
"Tool replacement and tool life diagnosis" in smart manufacturing is a key link between the quality of a workpiece and the efficiency of the manufacturing process; it is thus an indicator of the operating efficiency of machine tools. This technology combines on-site monitoring data, laboratory analysis and deep learning to provide best tool selection and processing of parameter combinations for different workpiece materials and production capacity situations, enhancing the effectiveness of machine tools.
In the machine tool industry, the traditional diagnosis of the manufacturing process is mainly based on human judgment and experience. Therefore, it is impossible to make accurate judgments in time, and there is also the possibility of human negligence, which can lead to misjudgments and loss of material/ tools. In worst cases, it may even cause damage to the machine tool, requiring a considerable amount of money and time for repair. In recent years, as the needs of customers have more customized requirements, the research and development of metal materials used by manufacturers has been changing rapidly. At the same time, experienced operators of machines are gradually ageing and facing the crisis of passing on their experience. As one of the widely used types of equipment in the manufacturing industry, machine tools must respond to "Lack of key data, relying on accumulation of experience," leading to the pain point of being unable to cope with changes in new materials, new tools, and production capacity requirements which cause challenges.
The global smart manufacturing trend is rising rapidly, and the trend of smart automation and smart factory applications will drive the growth of smart device demand. A major trend in the development of intelligent manufacturing in the industry is to use sensors as the main tool for monitoring, and then to conduct big data analysis as a basis for smart manufacturing decision-making. Our research team has long worked on machine tools. The R&D of the upstream and downstream ecosystems includes a complete tool study from tool manufacturing to process manufacturing, and collaborates with industry to develop key smart manufacturing technologies with academic energy. The technology adopted by leaders in the machine tool industry can now be used to evaluate tools’ lifespans in different cutting situations.
The composition of metal materials affects the machining process. For example, the carbon composition obviously affects the properties of steel. High-carbon steel has high strength and contains carbides that will wear cutting tools, making it difficult to process. On the contrary, low-carbon steel easily sticks to the cutting tool, which shortens the tool’s working life. In addition, although magnesium and nickel increase the strength and toughness of the steel, they also have negative effects on processing. The difficulty of cutting metal materials usually depends on: (a) the chemical composition of the working material, (b) the structure, (c) the mechanical properties, (d) the physical properties, and (e) the cutting conditions, so the content of chemical elements leads to metal materials causing ever-changing cutting properties of metal materials, and when the tools are gradually worn out with cutting, makes it difficult to predict practical processing. The research team has conducted online monitoring of cutting practices, and has established a cutting knowledge base with (a) cutting thrust and (b) motor current as the core, and has set up a laboratory analysis environment to collect (c) surface roughness, (d) chip characteristics. and ( e) the data related to cutting characteristics such as cutting temperature which are used as the verification of the diagnosis signal. Deep learning is introduced to predict the machinability of the material and to evaluate the difficulty of cutting metal materials. With the addition of emerging materials, it can quickly
match the suitable cutting parameters to accelerate the recognition of the difficulty of cutting new materials.
The quality of the product is determined by the dimensional accuracy and surface roughness. The factors that affect the surface roughness relate to the cutting conditions such as tool properties, processing parameters, workpiece properties, and cutting phenomena, shown as Fig. 1. Surface roughness is the "fingerprint" of the manufacturing process, so it is one of the effective tool life evaluation criteria, and has long been used in research. Our research team independently developed an embedded key load cell sensor. Through the research team’s design, in addition to having the advantage of stress measurement sensitivity and strain robustness, the waterproof and dustproof sensor assembly can ensure its safe use in the working environment where the cutting fluid is entrained with metal scrap flying in the repeated cutting of the tool. These economical sensors are used by domestic leading machine tool manufacturers to enhance the added value of high-end machines and the overall brand image. The cutting knowledge base established by this key monitoring technology is used on the standard machine, and the competitiveness of the standard machine is also enhanced. The tool warning function can be achieved through the motor’s current data of each machine. The accumulation of the database combined with deep learning technology can provide manufacturers with tool selection and optimized processing parameter combinations due to production capacity requirements and workpiece materials.
Fig. 1 On-line diagnosis system with band saw machine
STAY CONNECTED. SUBSCRIBE TO OUR NEWSLETTER.
Add your information below to receive daily updates.