In 2018, with 14.5% of its population over 65, Taiwan has become an aged society and is expected to become a super-aged society by 2026 (20.7%) according to official statistics (https://www.ndc.gov.tw/). With its remarkably aging population, Taiwan has to face the urgent challenge of how to efficiently apply its existent medical resources. As some surveys have revealed, the elderly generation is in desperate need of better medical treatment, rehabilitation and medical transportation services, which are therefore very important parts of the long-term care 2.0 plan.
Take the needs of patients with diabetes for example; these patients need transportation support to and fro among their habitation, care centers, and hospitals, during their routine visits for medical treatment. In the past, elderly patients were accompanied by caregivers, but the long transportation time may add uncertainties for each patient. This is a disturbing problem for the transportation staff to arrange schedules. As a result, the elderly are forced to spend much longer receiving medical treatment than necessary due to such transportation inefficiency, and the performance of the resource utilization of the long-term care fleet is also limited.
Improper scheduling plans for the patients, delays due to traffic jams, and inappropriate fleet routing therefore happen frequently. By introducing reinforcement learning techniques into the fleet scheduling and routing algorithm, the Q function was designed with consideration of the location of the elderly patients, the optimal route to medical treatments, the appointed time of the treatments, the congestion of the medical service in the medical institution, and the timely road conditions. Through the carpooling provided by dynamic scheduling, the average waiting time for medical treatments is thus expected to be shortened, and medical delivery tasks will likely be accomplished on time. In short, by applying artificial intelligence technology, the goal to make effective use of medical transportation services can be achieved, while the quality of medical delivery can be significantly improved.
In 2018, with 14.5% of its population over 65, Taiwan has become an aged society and is expected to become a super-aged society by 2026 (20.7%) according to official statistics (https://www.ndc.gov.tw/). With its remarkably aging population, Taiwan has to face the urgent challenge of how to efficiently apply its existent medical resources.
As some surveys have revealed, the elderly generation are in desperate need of better medical treatment, rehabilitation and medical transportation services, which are therefore very important parts of the long-term care 2.0 plan. Take the needs of patients with diabetes for example; these patients need transportation support to and fro among their habitation, care centers, and hospitals, during their routine visits for medical treatment. In the past, elderly patients were accompanied by caregivers, but the long transportation time may add uncertainties for each patient. This is a disturbing problem for the transportation staff to arrange schedules. As a result, the elderly patients are forced to spend much longer receiving medical treatment than necessary due to such transportation inefficiency, and the performance of the resource utilization of the long-term care fleet is also limited. Improper scheduling plans for the patients, delays due to traffic jams, and inappropriate fleet routing therefore happen frequently.
Reinforcement learning technology has enabled computers to beat professional champions of Go in recent years. In addition to playing Go to defeat humans, artificial intelligence technology should be applied in more areas to improve social welfare. By introducing the reinforcement learning technique into the fleet scheduling and routing algorithm, the Q function was designed with consideration of the location of the elderly patients, the optimal route to medical treatments, the appointed time of the treatments, the congestion of the medical service in the medical institution, and the timely road conditions. Through the carpooling provided by dynamic scheduling, the average waiting time for medical treatments is thus expected to be shortened, and medical delivery tasks will likely be accomplished on time. In short, by applying artificial intelligence technology, the goal to make effective use of medical transportation services can be achieved, while the quality of medical delivery can be significantly improved.
By design, the traffic data required for the fleet scheduling and routing algorithm will be computed by detecting cars in the images captured by the fisheye camera sets on the traffic signs at intersections. Compared with traditional digital cameras, the new fisheye cameras offer vision with a much more extensive range. One drawback of such vision is that the images can be distorted. To solve this problem, specialized convolution neural network models and algorithms have been designed for traffic estimation. In the future, integrated services combined with the smart medical transportation service and smart hospital systems will provide high-quality smart medical services, satisfying the necessity of the ultra-age society.
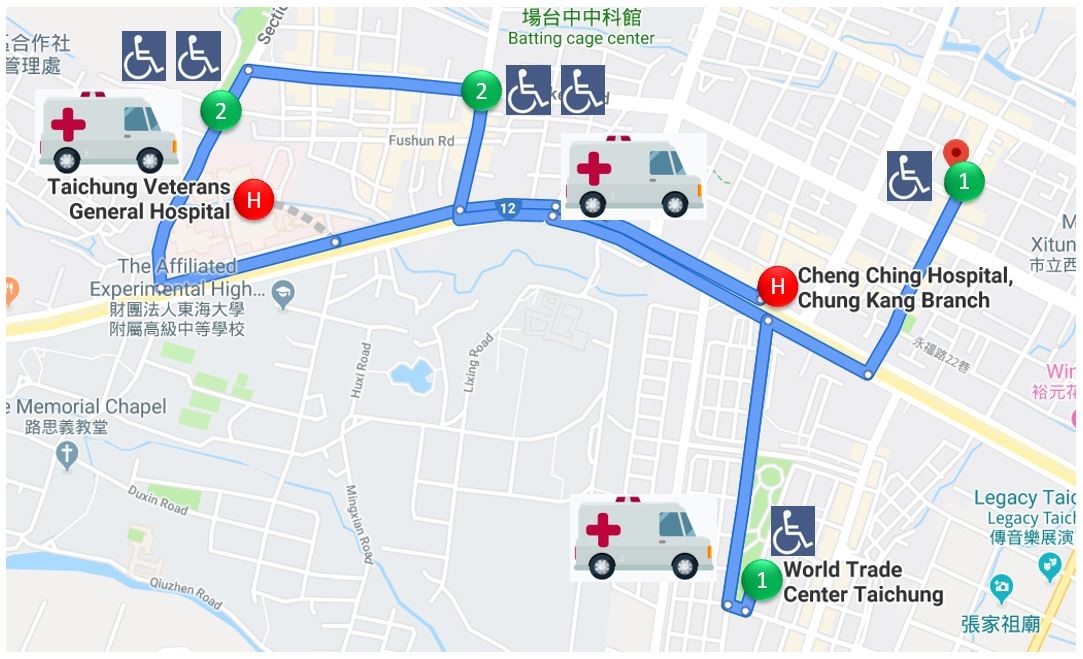