Privacy Protection and Personal Information De-Identification for Artificial Intelligence Applications
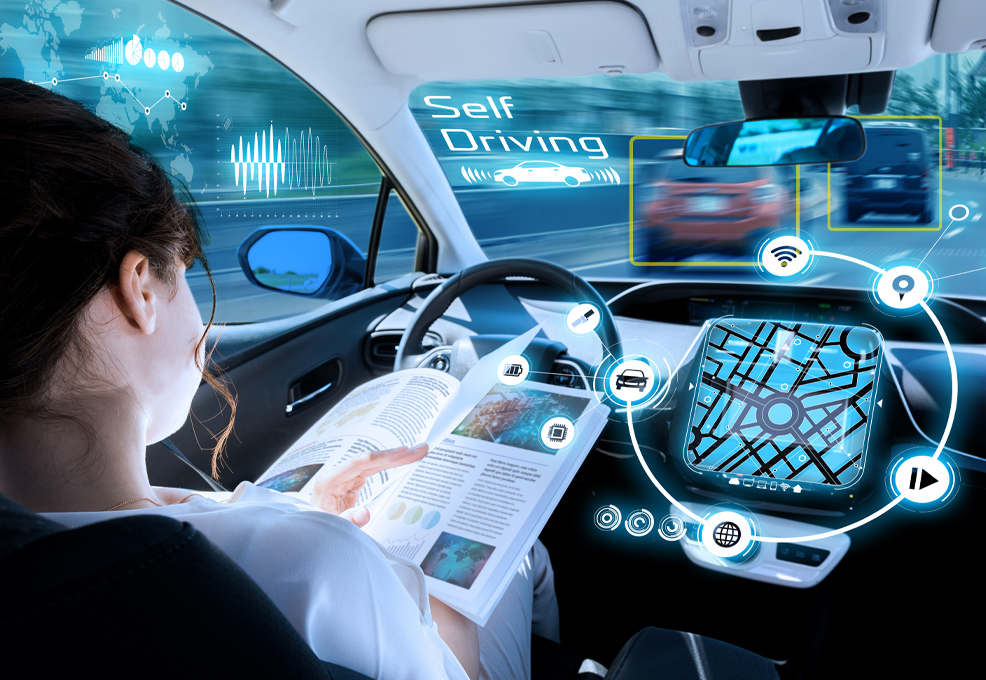
Author(s)
Jyh-Ching JuangBiography
Jyh-Ching Juang received his Ph. D. degree in electrical engineering from the University of Southern California, Los Angeles, CA, USA, in 1987. He is a Professor at the Department of Electrical Engineering, National Cheng Kung University, Taiwan. His research interests include autonomous driving, GNSS signal processing, and CubeSat design.
Academy/University/Organization
National Cheng Kung University-
TAGS
-
Share this article
You are free to share this article under the Attribution 4.0 International license
- ENGINEERING & TECHNOLOGIES
- Text & Image
- December 20,2019
The mobility of humans and goods is currently undergoing a paradigm shift towards autonomous, connected, electrified, and shared (ACES) driving. An ACES vehicle is equipped with all-around sensors such as cameras, radars, lidars, and thermal imagers to make intelligent decisions. The perception capability of sensor-based solutions is, however, limited in spite of the sensor fusion techniques that are used. Also, the processing complexity must be matched with appropriate processing power to achieve a balance in cost and performance. To increase the level of autonomy, a beyond-sensor-range solution is proposed. A two-way connectivity between vehicles and control center is envisioned in which vehicle-relevant information is uplinked to the control center for better monitoring and coordination, and dynamic traffic and map information is downlinked to the vehicles for safe and efficient operation. To achieve a responsive and trustworthy implementation, edge computation plays an essential role. The investigated edge computation is characterized by the software framework and, more importantly, an assessment of the real-time computational loads in autonomous driving. The connectivity and edge computation scheme was verified using National Cheng Kung University’s autonomous driving vehicle, and was tested at the Taiwan CAR laboratory. Future autonomous vehicles are required to operate with a guaranteed level of safety to be part of the smart city. The beyond-sensor-range solution implies that the right-of-way can essentially be claimed through information exchange in the virtual way, which in turn paves the way for the physical vehicle to move safely and efficiently in real-time.
In recent years, we have been witnessing a mobility transformation through the ACES technologies: autonomous, connected, electrified, and shared mobility. The availability of this transformation is expected to be beneficial to the society through the improvement of driving safety, enhancement of transportation efficiency, mitigation of the environmental impacts, and deployment of mobility as a service (MaaS). The ACES technology is also regarded as essential in dealing with an ageing society and the lack of professional drivers in some sectors, and will eventually be integrated into the digitized smart city. It is further noticed that when the eyes, hands, and mind are removed from the driving tasks, a passenger economy may arise and a new logistics system is needed. The above benefits have also transformed the industries in the sense that high-technology information companies, traditional car manufacturers, and innovative mobility service providers are cooperating and competing in the hope of sharing a portion of the market that is expected to be $173 billion in 2030 and $7 trillion by 2050.
The development of ACES technology and the deployment of ACES services are, however, considered to be very challenging. To realize Level 4/5 autonomous driving requires a system integration of different technical ingredients and a comprehensive sequence of tests to verify and validate the system. Traditionally, the enabling techniques are typically abbreviated as SPA, standing for Sense, Plan, and Act. Sense is related to the perception of the environment by processing and fusing sensor data. Plan is to perform the decision making and planning tasks so that collisions can be avoided, traffic rules can be observed, and the destination can be reached in a minimal-time or eco-friendly manner. Act is to control the vehicles to realize the sense and to plan results with small deviation, fast agility, and high stability. The operations of the Sense, Plan, and Act are essentially sequential. Typically, the sensor input of the processing chain is rich on data amount and high on data rate, while the control output stresses the accuracy, robustness, and trustworthiness, even when the output data are low in terms of rate and amount.
A high-level intelligent vehicle is required not only to perceive the current environment but also to predict the future situation in order to render a truly safe and efficient mobility. To this end, it is believed that in addition to SPA, additional C and E are needed, making SPACE the core enabling technology. C in the above stands for connectivity and E refers to edge computation. In the proposed solution, the vehicle-to-cloud or vehicle-to-control-center (V2C) capability is investigated. In this setup, the data being collected can be linked to the control center so that the status (position, battery management, and so on) of the vehicle and observations of its surrounding can be monitored and recorded. In addition, the control center can perform a global optimization based on real-time traffic information. The operation of high-level autonomous vehicles is often augmented with high-definition maps (HD-maps) which are known to be highly-accurate, rich-in-content, and dynamic. The data can then enhance the localization, perception, and guidance capability of the ACES vehicle. The operations about sensing, planning, action, and connectivity must be carried out in a responsive and trustworthy manner. Edge computation is needed to perform all the in-vehicle intelligent functions in real time and with limited resources. Even though edge computation may be picturized by a computer with gateways and graphical computer units, it is the software that is crucial to the successful autonomous functions. The proposed solution investigates the software framework and, more importantly, devises a method to assess the real-time computational loads in autonomous driving. Such a scheme is beneficial in designing a system with a balance of cost and performance.
The connectivity and edge computation scheme was verified using National Cheng Kung University’s autonomous driving vehicle, and was tested at the Taiwan CAR laboratory. As depicted, vehicle data are shared with the control center so that the status is monitored. As a result, the vehicle serves as a node in the internet of things of the overall mobility system to enhance cloud intelligence. The edge intelligence is also augmented through the connectivity as near real-time data can be brought to bear in intelligent decision making.
Future autonomous vehicles are required to operate with a guaranteed level of safety to be part of the smart city. The beyond-sensor-range solution implies that the right-of-way can essentially be claimed through information exchange in the virtual way which then paves the way for the physical vehicle to move safely and efficiently in real-time. The addition of the C and E elements to the SPA capability can potentially be used to enhance the level of automation of one vehicle. More importantly, through the collective effort of edge and cloud intelligence, the overall mobility system and service can be realized with a high level of safety and efficiency.
STAY CONNECTED. SUBSCRIBE TO OUR NEWSLETTER.
Add your information below to receive daily updates.