C-type Lectins in Host-Pathogens Interaction
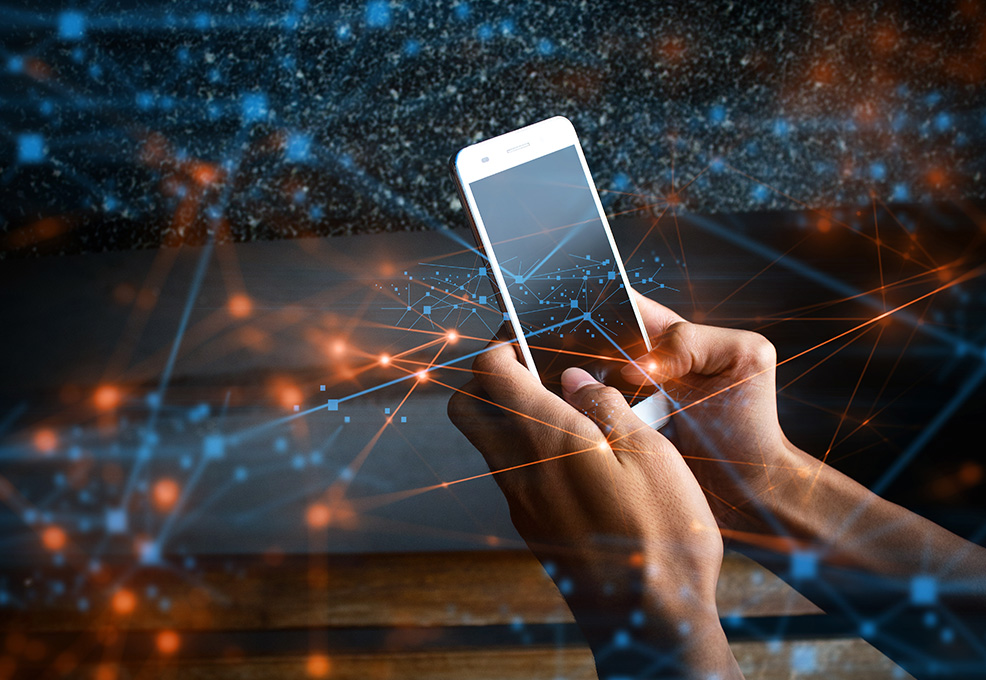
Author(s)
Der-Jiunn DengBiography
Der-Jiunn Deng received his Ph.D. degree in Electrical Engineering from National Taiwan University in 2005. He joined National Changhua University of Education as an Assistant Professor in the Department of Computer Science and Information Engineering in August 2005 and became a Distinguished Professor in August 2016. In August 2018, he was seconded to Overseas Chinese University as the Dean of Research and Development for a period of one year, and was then seconded to the Department of Industrial Technology, Ministry of Economic Affairs, as a Science and Technology Expert. Dr. Deng is an EAI fellow and has received a number of research awards.
Academy/University/Organization
Department of Industrial Technology, Ministry of Economic Affairs-
TAGS
-
Share this article
You are free to share this article under the Attribution 4.0 International license
- LIFE SCIENCES
- Text & Image
- March 17,2021
Since 2018, the Open Radio Access Network (O-RAN) has emerged as a new paradigm of mobile network infrastructures, and is projected to dominate the global market share of the fifth generation (5G) mobile networks from the year 2027 onwards. This is due to its cost efficiency, flexibility, and alleviation of vendor lock-in concerns, as compared with the conventional RAN. In addition to these merits, O-RAN reveals architecture sustaining artificial intelligent (AI) and/or machine learning (ML) algorithms, which enables a RAN to support any vertical application over any deployment scenario. This unprecedented capability therefore makes the integration of mobile network architectures with AI/ML computation an inevitable feature of the sixth generation (6G) mobile networks. In this article, we emphasize the integration of RANs and AI/ML and review the recent development of O-RAN. The inspiration for the design of 6G and challenges to the architecture of O-RAN are also examined.
With the recent development of artificial intelligent (AI) and machine learning (ML) algorithms, there have been manifold applications of AI/ML algorithms in different domains. This trend is projected to continue in the following decades. To sustain these applications, the sixth generation (6G) mobile networks should not only offer an enhancement of the International Mobile Telecommunications 2020 (IMT-2020) systems (also known as the fifth generation, 5G, mobile networks). Instead, the system architecture of 6G mobile networks should be integrated with AI/ML algorithms as a new sort of computing platform.
The interplay between AI/ML algorithms and 6G mobile networks can be twofold: 1) Regarding the use cases of AI/ML algorithms (for example, computer vision, pattern recognition, augmented/virtual reality, and so forth) as a class of services to be supported by 6G, 3GPP has evaluated the quality-of-service (QoS) requirements of these services. The corresponding communication/networking functions satisfying these requirements will be subsequently designed in the 6G mobile networks. 2) Unlike the IMT-advanced systems (also known as the fourth generation, 4G, mobile networks) which only support a single class of wireless services such as undiscriminating latency, reliability, and data rate optimization, the most remarkable feature of the IMT-2020 systems is their capability to support multi-class wireless services with different requirements in terms of latency, reliability, connection density, and data rates. This emerging feature, however, greatly increases the complexity of the designs, deployment and performance optimization for the radio access network (RAN) of 5G and 6G. When the RAN of 5G/6G has been developed by a vendor, this RAN is supposed to support manifold vertical applications of virtual/augmented reality, unmanned vehicles/robots, digital twins, remote healthcare, smart factories, and so forth, with different requirements. To satisfy the requirement of a certain vertical application, a RAN should search for the most appropriate transmission function/scheme among all the supported functions/schemes; however, this depends on the practical deployment scenario (for example, indoor/outdoor, mobile/fixed, type of devices, sub 6 GHz or mmwave, shared/unshared core networks, and so forth). As a result, a RAN should learn the communication environment and the deployment scenario, so as to optimize the performance for any vertical applications. To address these engineering concerns, AI/ML algorithms have been regarded as an effective means to optimize the performance of any vertical application over any deployment scenario.
The global industry (especially the operators) has achieved consensus on two core principles to evolve to the next generation RAN: 1) openness and inter-operability: to attract more participation and collaboration of vendors in the designs and implementations of RAN; and 2) intelligence: to enable AI/ML in RAN for autonomous deployment and performance optimization. To this end, the Open RAN (O-RAN) Alliance was established in 2018, to offer a “white box” solution of RAN. The O-RAN Alliance is now composed of 27 operator members and more than 200 contributors. The paradigm shift of mobile network infrastructures is illustrated Figure 1.
Fig. 1. The paradigm shift of mobile network infrastructures.
To achieve openness, the functions of RAN in the O-RAN are virtualized and divided into four key units: (i) The Radio Unit (RU) embraces the radio frequency (RF) components and lower-physical layer. (ii) The Distributed Unit (DU) sustains the higher-physical layer, medium access control (MAC) layer and radio link control (RLC) layer. (iii) The Central Unit (CU) hosts the radio resource control (RRC) protocol, packet data convergence protocol (PDCP) and service data adaptation protocol (SDAP). (iv) Most importantly, the RAN Intelligent Controller (RIC) is unprecedentedly introduced to accommodate ML algorithms. Interfaces to connect these four units are also standardized, including A1, O1, E1, E2, F1 and open fronthaul. On the other hand, to achieve intelligence, various ML algorithms can be applied to the Near Real-Time (Near-RT) RIC collocated with RAN and Non-Real-Time RIC (Non-RT) installed in Service, Management and Orchestration (SM&O) toward predictive and/or optimal resource/communication configuration for RANs. The architecture of a RAN as per O-RAN is illustrated in Figure 2.
Fig. 2. Architecture of a RAN as per O-RAN
RICs are the revolutionary units to enable ML algorithms in RAN. In the architecture of O-RAN, different ML algorithms are deployed in the form of “xAPPs” and “rAPPs” in the Near-RT RIC and Non-RT RIC, respectively. With different duty cycles of control to RIC (10-1000 ms in Near-RT RIC and several seconds in Non-RT RIC), the Non-RT RIC is responsible for model training, experience aggregation, and policy creation, while Near-RT RIC is responsible for machine inference (MI) based on the policy/model provided from Non-RT RIC, experience/model transfer to Non-RT RIC, and/or model training.
Although O-RAN reveals an initial framework of intelligent RANs, there are still a number of challenges in the practical deployment. Firstly, since the functions of RAN are divided into four units, the performance and operation reliability/availability may be a concern. Secondly, there may be multiple ML algorithms to be installed in the Near-RT RIC and Non-RT RIC, and these ML algorithms may interact with each other. Additional designs may therefore be required for the orchestration of these ML algorithms. Thirdly, the energy consumption of the 5G RANs has been a critical burden. To further sustain ML algorithms, platforms with powerful computing capabilities may be required, which may cause more energy consumption. This may not be desirable for small cell deployment.
STAY CONNECTED. SUBSCRIBE TO OUR NEWSLETTER.
Add your information below to receive daily updates.