A Fire in Your Body: Inflammation Is at the Root of Most Diseases
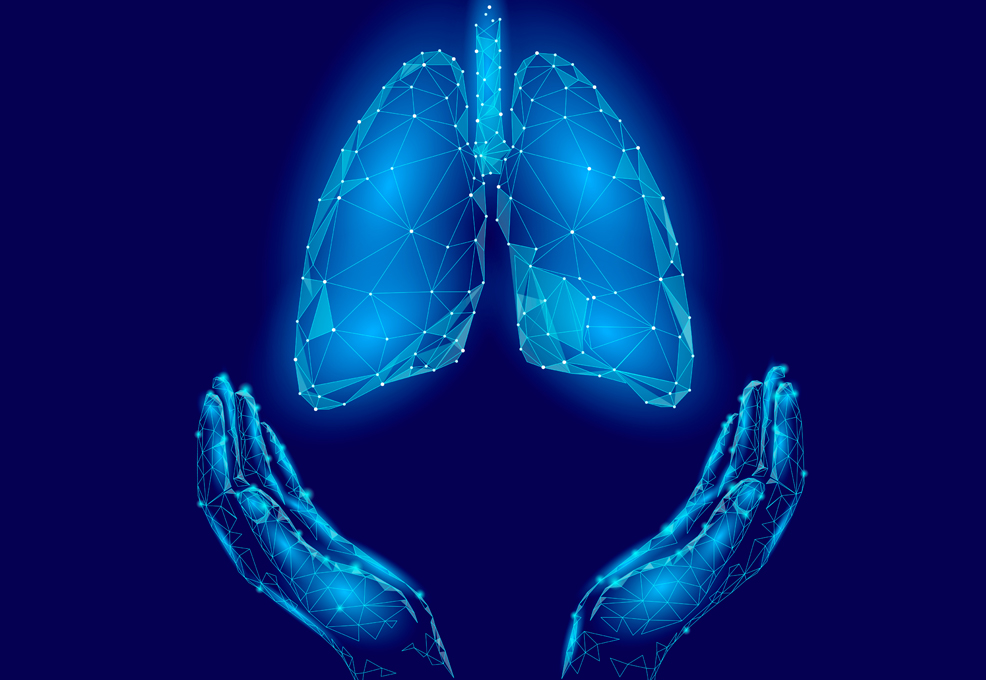
Author(s)
Yung-Nien SunBiography
Director of MOST AI Biomedical Research Center in National Cheng Kung University (NCKU). Distinguished prof. at the Department of Computer Science and Information Engineering of NCKU.
Academy/University/Organization
National Cheng Kung UniversityEdited by
NCKU group-
TAGS
-
Share this article
You are free to share this article under the Attribution 4.0 International license
- LIFE SCIENCES
- Text & Image
- February 19,2019
According to the WHO 2018 report, Tuberculosis (TB) is one of the top ten causes of death worldwide, and it is the leading cause of a single infectious agent (over HIV). Globally, 10 million cases developed in 2017 and 1.3 million deaths resulted from it. It is a global infectious disease dilemma, especially in undeveloped and developing countries. WHO has announced an End TB Strategy and the goal is to end the TB pandemic by 2030.
Numerous evaluation methods are available for TB, such as medical history, a physical examination test for MTB infection, chest radiographs, and bacteriologic examination of clinical specimens. Among these, visual tests such as chest radiographs and acid-fast smear bacteriologic classification are widely applied. However, a critical problem of the current visual diagnosis is the drain on professional manpower. Therefore, an automatic, fast and accurate MTB identification system is highly desirable. In recent years, AI has made an important breakthrough. The deep learning convolution neural network (CNN) shows amazing performance on machine vision tasks which can be applied to the visual diagnosis to help the fight against TB.
System platform
Identification result
According to the WHO 2018 report, Tuberculosis (TB) is one of the top ten causes of death worldwide, and it is the leading cause of a single infectious agent (over HIV). Globally, 10 million cases developed in 2017 and 1.3 million deaths resulted from it. It is a global infectious disease dilemma, especially in undeveloped and developing countries. WHO has announced an End TB Strategy and the goal is to end the TB pandemic by 2030.
TB is an airborne disease caused by Mycobacterium tuberculosis (MTB). MTB usually affects the lungs, and can affect other regions of the body as well. Someone infected by pulmonary MTB can easily transfer the disease through the air in public areas. A fast and accurate diagnosis can help patients get treatment sooner and prevent the spread of the disease.
Numerous evaluation methods are available for TB, such as medical history, a physical examination test for MTB infection, chest radiographs, and bacteriologic examination of clinical specimens. Among these, visual tests such as chest radiographs and acid-fast smear bacteriologic classification are widely applied. However, a critical problem of the current visual diagnosis is the drain on professional manpower. Therefore, an automatic, fast and accurate MTB identification system is highly desirable.
In recent years, AI has made an important breakthrough. The deep learning convolution neural network (CNN) shows amazing performance on machine vision tasks which can be applied to the visual diagnosis to help the fight against TB.
CNN performs the machine vision task end-to-end, where the test image can be directly input into the network and the prediction result will be obtained. It overcomes the disadvantage of complicated processes of conventional methods, and improves accuracy. As AI techniques advance, machine learning has become a key approach to solving problems. The machines will become more intelligent by learning more data. For this mission, the CNN is a powerful technique, and the digital smear microscopy images and chest radiographs are the data.
The AI diagnosis system can be applied globally. Users can access the system through the Internet and can download it to a computer. The CNN is trained with a huge and continuously growing number of images. After taking and inputting the digital images of sputum smears or chest radiographs, one can easily and quickly retrieve the assisted diagnosis results from the system.
Recently, Prof. Yung-Nien Sun at the Ministry of Science and Technology (MOST) AI Biomedical Research Center has been leading a project to develop a system for automatic TB identification from acid-fast stained sputum smear specimens. The deep learning CNN technique has been designed to detect and identify MTB. The system is equipped with a patented automatic image acquisition technique and provides outstanding TB identification results. A given example showed that the proposed identification system highlights the detected MTB with a bounding box and a confidence score representing the probability of MTB. There is also a database with more than 50,000 smear microscopy images constructed for the training and evaluation of the identification system. The sensitivity is 95% and the specificity is over 94%. The system has achieved remarkable results on blind tests of TB smear identification from several medical centers in Taiwan. The system method and preliminary experiment results were published in an international journal and a patent was applied for.
Besides sputum microscopy, numerous AI systems for chest radiography have also been developed globally. Software called CAD4TB was designed using machine learning techniques to obtain higher accuracy and faster processing in X-ray TB detection. The system is trained using thousands of healthy and diseased X-ray data from over 15 countries. The system is equipped to detect TB by a score and heat map for the assistance of diagnosis.
Another application worth speaking of is SemanticMD AI Box, a portable, low-cost, accessible TB detection device which can analyze a chest X-ray within 20 seconds. Deep learning technologies are applied in the box, and the algorithm is trained on a growing database of thousands of images from different countries. The input is the digital X-ray data, and the box supports DICOM and HL7 standards.
To fight against TB, there is still a long way to go. However, by employing the powerful and promising deep learning CNN methods, we are one more step closer to the goal of eradicating this disease. This is a primary step in the development of AI computer assisted diagnosis (AICAD) which is expected to achieve more breakthroughs.
STAY CONNECTED. SUBSCRIBE TO OUR NEWSLETTER.
Add your information below to receive daily updates.