DNA Biopolymer Photonics:An Innovative Platform Toward Sustainable Technology
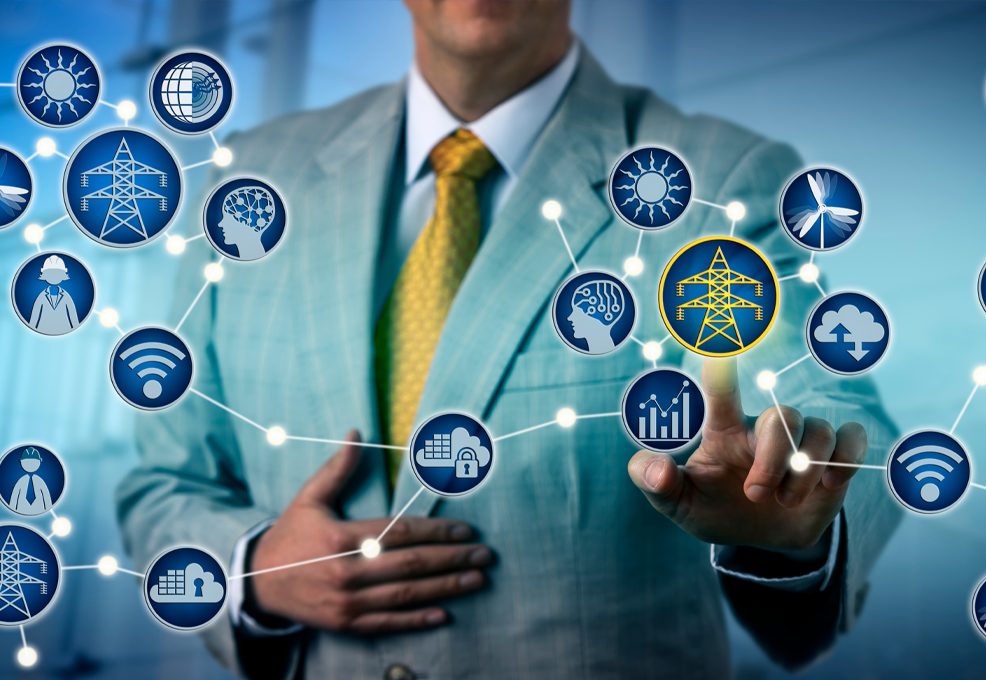
Author(s)
Faa-Jeng LinBiography
Faa-Jeng Lin is a Chair Professor at the Department of Electrical Engineering, National Central University, Taiwan. His research interests include AC motor drives, power electronics, renewable energies, smart grids, intelligent and nonlinear control theories. Several of his papers have helped to establish research areas such as fuzzy neural network control of motor drives and motion control systems, and resonant converters for piezo-ceramic motor drives. He was the President of the Taiwan Smart Grid Industry Association and the chair and principle investigator of the Smart Grid Focus Center, National Energy Project Phases I and II in Taiwan. He is now the Executive Director of the Taiwan Power Company. Professor Lin received the Outstanding Research Awards from the National Science Council, Taiwan, in 2004, 2010 and 2013. He is also an IEEE Fellow.
Academy/University/Organization
National Central UniversitySource
K. H. Tan, F. J. Lin, C. M. Shih, and C. N. Kuo, “Intelligent Control of Microgrid with Virtual Inertia Using Recurrent Probabilistic Wavelet Fuzzy Neural Network,” IEEE Trans. Power Electronics, accepted, 2019. (SCI, IF 7.224, 16/266) MOST 108-3116-F-008-001-
TAGS
-
Share this article
You are free to share this article under the Attribution 4.0 International license
- ENGINEERING & TECHNOLOGIES
- Text & Image
- March 20,2020
A microgrid with virtual inertia using master-slave control is proposed in this study to overcome the drawbacks of traditional inverter-based distributed generators for lack of inertia and without grid-forming capability. The microgrid using master-slave control is composed of a storage system, a photovoltaic (PV) system and a varying resistive three-phase load. The storage system and PV system are regarded as the master unit and the slave unit respectively in the microgrid. Moreover, in order to improve the reactive power control in grid-connected mode and the transient response of the microgrid during the switching between the grid-connected mode and the islanding mode, an online trained recurrent probabilistic wavelet fuzzy neural network (RPWFNN) is proposed to replace the conventional proportional-integral (PI) controller in the storage system. Furthermore, when the microgrid is operated in islanding mode, the load variation will have a serious influence on the voltage control of the microgrid. Thus, the RPWFNN control is also proposed to improve the transient and steady-state responses of voltage control in the microgrid. Finally, according to some experimental results, excellent control performance of the microgrid with virtual inertia using the proposed intelligent controller can be achieved.
Nowadays, due to the growing awareness of the energy crisis and environmental concerns, the penetration of distributed generations (DGs) using renewable energy sources has been significantly increased in the power system. Thus, a novel concept of the microgrid, which integrates the loads and the DGs at the customer side and can be operated in either grid-connected mode or islanding mode, has arisen in the power system. The cover photo is the first microgrid demonstration site in Taiwan which was built in the Institute of Nuclear Energy Research (INER). The microgrid is regarded as a small-scale power system and can improve grid performance and provide flexibility to its operation. The algorithms of the DGs in the microgrid can be generally divided into two categories: master-slave control and droop control. The main purpose of the master-slave control is to ensure the outputs of the DGs of the microgrid to track the active/reactive power commands or voltage/frequency commands. One of the DGs is regarded as the master unit and is operated under the active/reactive power control in grid-connected mode. When the microgrid is operated in islanding mode, the master unit is switched from the active/reactive power control to the voltage/frequency control to maintain the voltage and frequency of the microgrid. The rest of the DGs are regarded as the slave units and are operated under the active/reactive power control in both grid-connected mode and islanding mode.
Conventional grid-connected power converters are normally operated as current sources and have no frequency regulation capability. Therefore, the power system will be unstable as a result of the lack of rotating inertia if a large amount of inverter-based DGs are deployed. In other words, the dynamic responses of the inverter-based DGs are much faster than those of the conventional synchronous generator (SG) which will make the power system susceptible to oscillation resulting from sudden load variations or external disturbances. Owing to the merit of robustness of the rotating inertia, inverter-based DGs using a virtual synchronous generator (VSG) was developed in this study to emulate the virtual inertia by using the essential swing equation to mimic the transient characteristics of SG.
An online trained recurrent probabilistic wavelet fuzzy neural network (RPWFNN), which comprises the merits of the inference capability of the fuzzy neural network (FNN), the approximation accuracy of the wavelet neural network (WNN), the random processing ability of the probabilistic fuzzy neural network (PFNN) and the internal dynamic capability of the recurrent neural network (RNN), is proposed in this study to improve the control performance of the microgrid. Therefore, in this study, a microgrid with virtual inertia using the master-slave control algorithm was developed to overcome the drawbacks of inverter-based DGs which lack rotating inertia and do not have grid-forming capability. In addition, the intelligent RPWFNN controller is proposed to replace the conventional proportional-integral (PI) controller in the storage system to improve the reactive power control performance in grid-connected mode and the transient response during mode switching.
Figure 1 shows the grid-connected mode of the microgrid. In Figure 1, is the active power output;
is the reactive power output of the storage system;
is the active power command of the storage system;
is the active power command of the storage system. The q-axis control current for the active power control is generated by a PI controller. Meanwhile, the d-axis control current for the reactive power control is obtained through a PI or the proposed RPWFNN controller using the reactive power error as input. When the power grid fails, the microgrid can immediately disconnect from the power grid via the SSR and smoothly switch from the active/reactive power control to the voltage/frequency control as shown in Figure 2. In Figure 2, the peak value of the a-phase voltage
and the frequency
of the microgrid are compared with the peak value of the phase voltage command
and the frequency command
. Thus, the q-axis control current for the voltage control is obtained through a PI or the proposed RPWFNN controller. Meanwhile, the d-axis control current is obtained through the swing equation and a PI controller for maintaining the frequency of the microgrid. Finally, the control signals are calculated through coordinate transformation with the angle command
to obtain the SPWM switching signals for the storage system operated in islanding mode.
Prof. Lin has developed and transferred some microgrid technologies to several companies in Taiwan. For instance, the photovoltaic (PV) and battery energy storage system management system was transferred to NextDrive Inc., Taiwan, in 2019; the active filter algorithm for microgrids was transferred to Chung-Hsin Electric Machinery Mfg. Inc. in 2017; the low voltage ride-through technology for the grid-connected PV power plant was transferred to Controlnet International Inc., also in 2017. According to the databases of IEEE Xplore and Thomson Reuters ISI Web of Science, Prof. Lin is the pioneer in applying fuzzy neural networks to the grid-connected control of PV systems.
STAY CONNECTED. SUBSCRIBE TO OUR NEWSLETTER.
Add your information below to receive daily updates.