The Mission of Oncologists-from Cancer Research to Precision Medicine
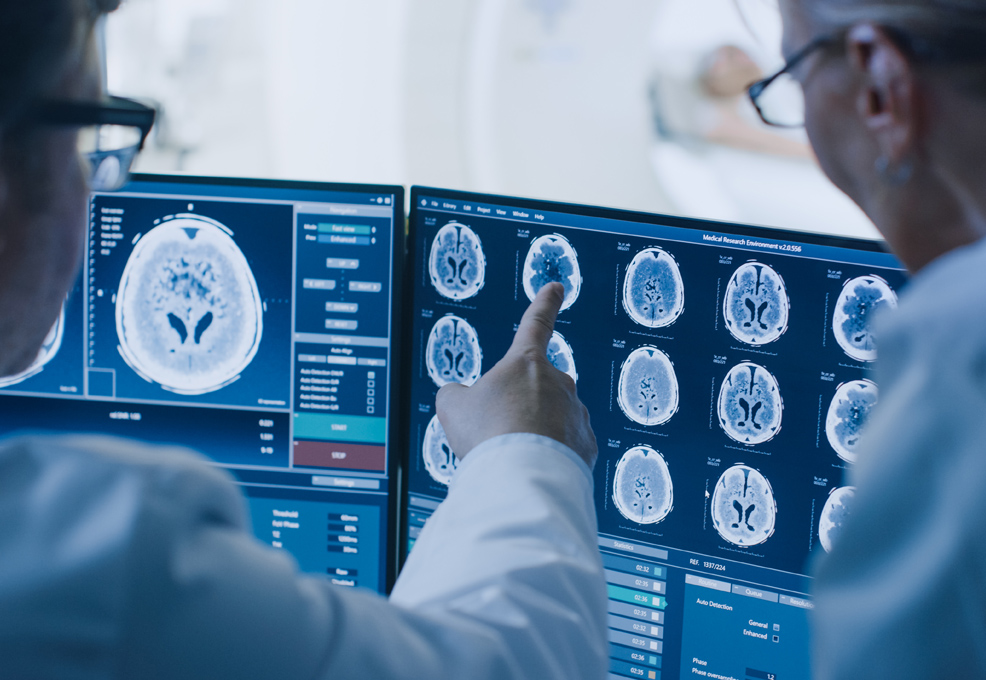
Author(s)
Cheng-Yu ChenBiography
Dr. Cheng-Yu Chen is a board-certified neuroradiologist and a renowned scientist in the field of brain tumors, mild traumatic injury and stroke. He is also the vice president of Taipei Medical University.
Academy/University/Organization
Taipei Medical UniversitySource
Machine Learning–Based Radiomics for Molecular Subtyping of Gliomas. Clin Cancer Res. 2018 Sep 15;24(18):4429-4436. DOI: 10.1158/1078-0432.CCR-17-3445. Epub 2018 May 22. https://clincancerres.aacrjournals.org/content/24/18/4429.long-
TAGS
-
Share this article
You are free to share this article under the Attribution 4.0 International license
- LIFE SCIENCES
- Text & Image
- September 25,2019
Glioblastoma (GBM) is among the deadliest forms of brain cancer. Even after decades of basic and clinical research, the therapeutic outcome of GBM patients remains feeble, with an overall survival time ranging from 12 to 18 months, and less than 5% of patients surviving longer than five years after diagnosis. Recent advances in the understanding of genetic and molecular mechanisms of GBM have opened new opportunities in GBM treatment. Big data analysis reveals strong survival correlation in GBM patients harboring IDH1 gene mutation and 1p and 19q co-deletion; GBM with methylated DNA repaired genes were less resistant to standard chemotherapy. On the other hand, the immune status within and surrounding the GBM may also affect the new choice of treatment such as the check point inhibitors. We recently developed a non-invasive MRI technique, known as radiogenomics, which can decode the genetic mutations through imaging texture analysis (the radiomics). This new Omics algorithm can extract information not available to the naked eye. Using machine learning technology, we can classify GBM MRI into five major molecular subtypes which further enhance the individual choice of treatment. Furthermore, with the same technique, the GBM micro-environment that encompasses important information regarding cancer immune status can be derived from the MR images. We are currently developing new artificial intelligence algorithms that may help GBM patients in personalized care, and hopefully improve their survival rate.
You have probably heard about how new target therapies or immunotherapy can dramatically cure certain cancers such as lymphoma, melanoma and leukemias. Nonetheless, those new therapeutic effects are apparently not transferable to the glioblastomas (GBM) which are the most common malignant brain tumors that always recur and have the shortest survival period, even after 40 years of vigorous research. The key problem is the genetic and biological heterogeneity of the GBM that produce tremendous hurdles in therapeutic strategies, as the cancers can adapt themselves to resist treatment. This leads to a critical thought regarding whether we can have a non-invasive technique that can single out GBM patients to get the most personalized treatment by showing the key gene mutations and relevant immune status of the cancer. In the last three years, with the funding of the Ministry of Science and Technology, Taiwan, Republic of China, we have developed new algorithms that can predict gene mutations and the immune status of cancer by employing feature extraction from MRI (Figure A).
Current diagnostic MRI can provide morphological and functional information regarding the GBM using state-of-the-art technology and high field strength MRI machines. That information is assessed by the radiologists and neurosurgeon to decide the treatment. For the genetic mutation data, fresh tumor tissue is required for high throughput analysis. The problems are the relatively high cost and the fact that processing is not timely for surgical planning. To solve the problem, we extracted thousands of features from hundreds of GBM images along with their gene profiles from “The Cancer Genome Atlas” (TCGA) and analyzed the big data using machine learning methods. After validating the algorithms we developed from TCGA using our local patient data, we were able to accurately predict five important molecular subtypes of GBM according to their gene mutations (for example, IDH1 mutation and 1p19q co-deletion) and survival, i.e. we can tell which GBM patient can respond better to treatment and thus survive longer. The point is that we can provide future GBM patients with the above-mentioned information just by MRI alone before surgical and chemotherapeutic planning. Figure B shows the comparable accuracy of our MRI prediction technique (radiogenomics) with respect to the gold-standard results from histological and genomic classification.
While the GBM patient outcome is mostly based on their genetic mutation profile (those with IDH1 mutation have better overall survival), focus has not been on those with IDH wild type (those patients have the worst prognosis). We are now working on assessing the immune status inside the GBM and their peri-tumoral regions (cancer microenvironment) by the same radiogenomic technology. The preliminary results reveal several interesting findings that MRI radiomic features can imply, including the cytotoxic T lymphocyte (CTL) expression status and types of macrophages which activate or suppress CTL. CTL is the final defense line from our body to clean the residual cancer cells. If we can quantify those CTL inside the solid part of GBM and the tissue surrounding them, we can probably apply new immunotherapy (such as immune check point inhibitors) to those patients selected by our new technique, in addition to classic therapy. This may individualize therapeutic strategies for GBM. Last but not the least, since our lab has scaled up the computing and analyzing power to machine learning, our algorithms can be industrialized to add to the clinical workflow in the future.
STAY CONNECTED. SUBSCRIBE TO OUR NEWSLETTER.
Add your information below to receive daily updates.