Moving Your Body, Strengthening Your Brain
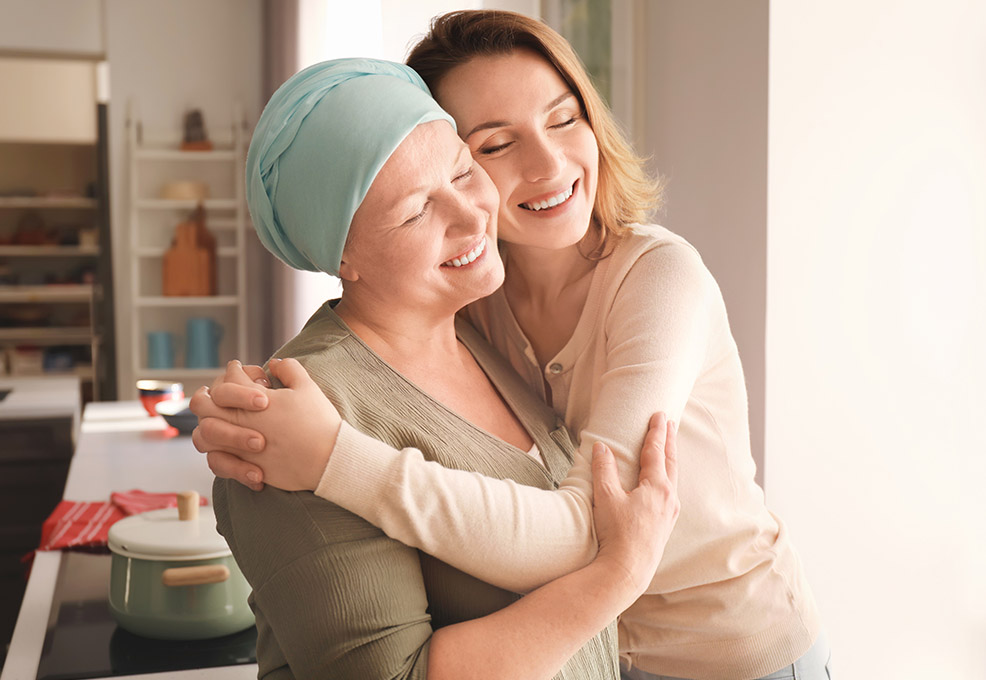
Author(s)
Shinn-Ying HoBiography
Dr. Shinn-Ying Ho is currently a professor and chairman of the Institute of Bioinformatics and Systems Biology at National Chiao Tung University (NCTU). He is also the founder of the research service company (Doctor How Inc.), which is a derivative startup of NCTU.
Academy/University/Organization
National Chiao Tung UniversitySource
1.Identification and characterization of the lncRNA signature associated with overall survival in patients with neuroblastoma, Scientific Reports volume 9, Article number: 5125 (2019)
https://www.nature.com/articles/s41598-019-41553-y
2.Identifying a miRNA signature for predicting the stage of breast cancer, Scientific Reports volume 8, Article number: 16138 (2018)
https://www.nature.com/articles/s41598-018-34604-3
3.Identifying the miRNA signature associated with survival time in patients with lung adenocarcinoma using miRNA expression profiles, Scientific Reports volume 7, Article number: 7507 (2017)
https://www.nature.com/articles/s41598-017-07739-y-
TAGS
-
Share this article
You are free to share this article under the Attribution 4.0 International license
- LIFE SCIENCES
- Text & Image
- June 18,2019
How long can cancer patients live? The prospect of survival for cancer patients depends on some key factors, such as the age at diagnosis, cancer type, cancer stage, cancer metastasis, and genetic features. As a result, the signature identification of biomarkers, such as genes and non-coding RNAs (ncRNAs), associated with survival time is urgently required for precision medicine. A biomarker signature is defined as a minimal subset of biomarkers that are maximally predictive with respect to survival time when they work cooperatively. Biostatistics approaches are commonly used for discovery of individual biomarkers, while machine learning approaches are appropriate for identifying biomarker signatures and establishing prediction models from a large dataset, e.g., expression profiles of genes/ncRNAs. However, the training expression profiles of cancer patients are usually characterized by high-dimensional samples and small datasets, which result in both curse of dimensionality and underdetermined problems.
We propose a novel evolutionary learning platform on complex biomedical data for identification of biomarker signatures while considering the underdetermined problem. For example, the proposed method identified 18 out of 332 miRNAs and achieved a correlation coefficient of 0.88 ± 0.01 and mean absolute error of 0.56 ± 0.03 years between real and estimated survival time of lung adenocarcinoma patients. The platform has been validated using miRNA/lncRNA expression profiles of patients with lung adenocarcinoma, glioblastoma multiforme, breast cancer, ovarian cancer and neuroblastoma from The Cancer Genome Atlas and Gene Expression Omnibus databases. The analysis of all signatures of genes/ncRNAs for cancer patients can help to develop drugs and gene targeted therapy.
How long can cancer patients live? The prospect of survival for cancer patients depends on some key factors, such as the age at diagnosis, cancer type, cancer stage, cancer metastasis, and genetic features. Precision medicine plays multiple roles in improving the long-term survival of patients. As a result, the identification of biomarkers associated with survival time is urgently required for precision medicine. Recent advances in next-generation sequencing and microarray technologies have resulted in great interest in non-coding RNAs (ncRNAs), including small non-coding RNAs, such as miRNAs and piRNAs, and long non-coding RNAs (lncRNAs), which have shown their significant roles in various cancers. In particular, the role of ncRNAs in evolution and genome functions is a new topic of interest in cancer research. A biomarker signature is defined as a minimal subset of biomarkers (e.g., ncRNAs) that are maximally predictive with respect to survival time when they work cooperatively. Generally, machine learning methods emphasize predictive results and statistical methods care about causal reasoning. Biostatistics approaches are commonly used for discovery of individual biomarkers, while machine learning approaches are appropriate for identifying biomarker signatures and establishing prediction models from a large dataset (e.g., expression profiles of ncRNAs).
The evolutionary learning platform can identify various biomarker signatures of genes/ncRNAs associated with survival time, cancer stage and cancer metastasis from the datasets of expression profiles in patients with cancers. Using the feedback mechanisms of the growing datasets (e.g., TCGA and GEO), the AI-based platform can incrementally refine the identified signatures and the derived prediction models. The identified signature for designing prediction models can comprise not only genes/ncRNAs but also biochemistry values and lifestyle factors for precision medicine and healthcare. The biomarker signatures could aid in the development of novel therapeutic approaches to the treatment of various cancers. Furthermore, the combined analysis of all signatures of genes/ncRNAs with their related pathways for cancer patients can help to develop drugs and gene targeted therapy.
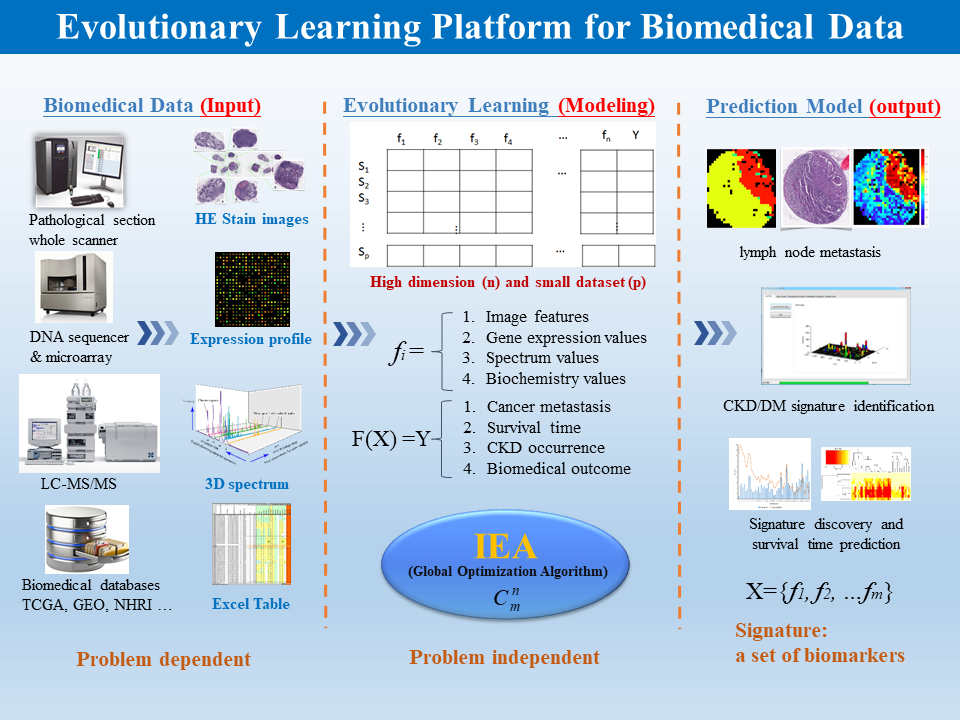
Figure 1: Evolutionary learning platform for biomedical data.
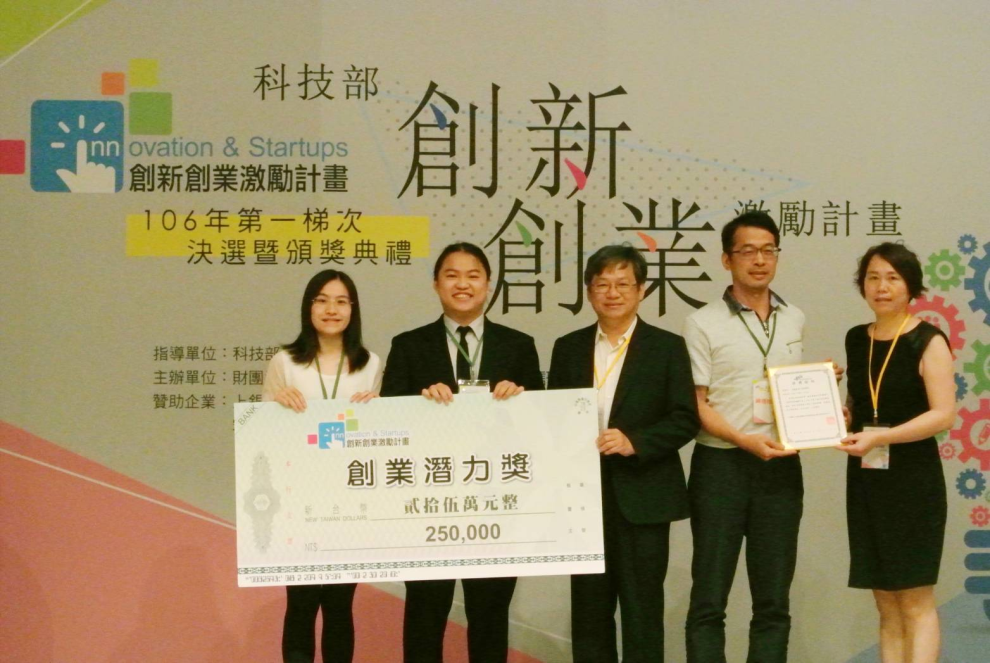
Figure 2: The computer aided diagnosis system won an Entrepreneurial Potential Award in the FITI Program Competition of the Ministry of Science and Technology.
STAY CONNECTED. SUBSCRIBE TO OUR NEWSLETTER.
Add your information below to receive daily updates.