Taiwan to Venture Into the Sea of Stars
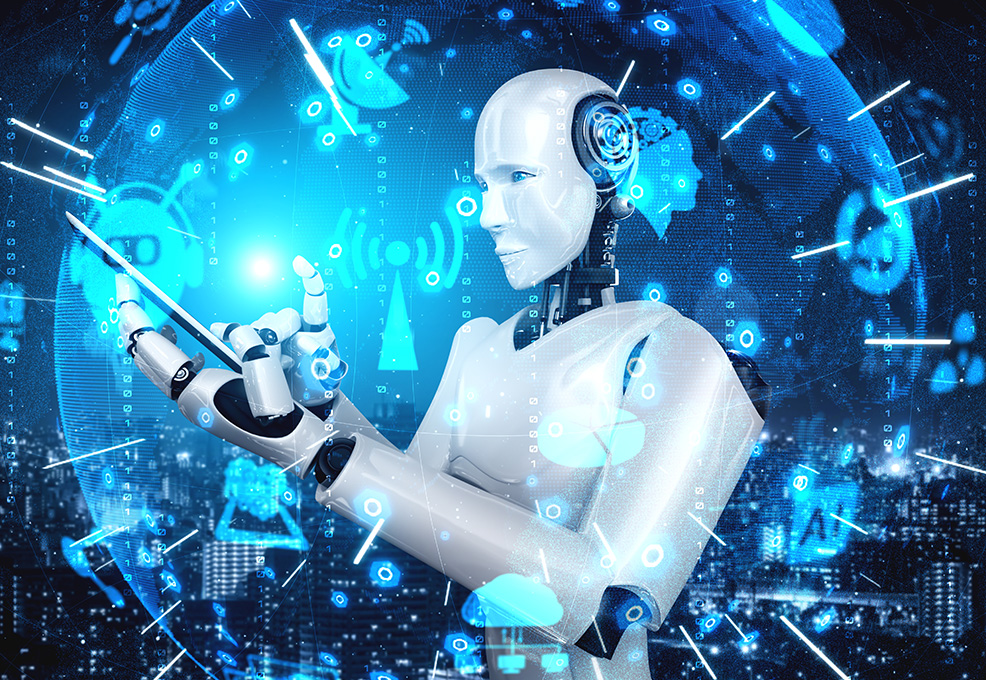
Author(s)
Chen-Fu ChienBiography
Dr. Chen-Fu Chien is Tsinghua Chair Professor & Micron Chair Professor, National Tsing Hua University, Taiwan. He is President of the Chinese Institute of Industrial Engineers, the Director of Artificial Intelligence for Intelligent Manufacturing Systems (AIMS) Research Center, MOST, Taiwan. He has been in the top 2% of international scholars according to Stanford research published in PLoS Biol 18(10): e3000918. doi:10.1371/journal.pbio.3000918
Academy/University/Organization
National Tsing Hua UniversitySource
Artificial Intelligence for Intelligent Manufacturing Systems (AIMS) Research Center, Ministry of Science & Technology, Taiwan.-
TAGS
-
Share this article
You are free to share this article under the Attribution 4.0 International license
- ENGINEERING & TECHNOLOGIES
- Text & Image
- April 14,2021
Driven by Moore’s Law, semiconductor manufacturing is becoming increasingly complicated due to continuous migration of advanced technologies for manufacturing excellence. As critical dimensions of integrated circuits are shrinking for nano technologies, we have developed interrelated solutions for yield enhancement and smart manufacturing that have been validated with empirical studies in leading semiconductor manufacturing companies. Focusing on realistic needs, novel solutions with the data-driven UNISON framework for advanced quality control (AQC), advanced process control (APC), and advanced equipment control (AEC) have been developed on the basis of leading technologies such as big data analytics, artificial intelligence, Run-to-Run (R2R) control, virtual metrology (VM), fault detection and classification (FDC), and Prognostics and Health Management (PHM). The results of empirical studies have shown the practical viability of the proposed solutions. Indeed, the developed solutions have been implemented in real settings. The paradigm of global manufacturing is shifting as leading nations propose the next phases of industrial revolution such as Industry 4.0. Alternatively, I proposed Industry 3.5 as a hybrid strategy to address fundamental objectives for smart manufacturing for those who may not be able to migrate to Industry 4.0 directly. Furthermore, semiconductor manufacturing should become the keystone of the global manufacturing ecosystem and the leader to address sustainable growth and the circular economy. Sponsored by the Ministry of Science & Technology, Taiwan, Artificial Intelligence for Intelligent Manufacturing Systems Research Center (AIMS) and my associates have been conducting more research and development to empower Industry 3.5 for Taiwan smart manufacturing to humanize the ongoing industrial revolution and share our experiences to support emerging countries.
Semiconductor manufacturing is capital intensive in which yield enhancement, manufacturing excellence, cycle time reduction, capital effectiveness, and cost reduction are crucial for maintaining competitive advantages. Driven by Moore’s Law, semiconductor manufacturing continuously migrates to advanced technology nodes by shrinking the critical dimensions of integrated circuits (IC). Overall wafer effectiveness (OWE) was proposed as a novel standard to measure wafer exposure effectiveness and derive effective solutions to optimize productivity and yields while minimizing overall cost.
Indeed, the semiconductor manufacturing industry has strived to address each of the factors to enhance quality performance. As critical dimensions of integrated circuits are shrinking for nano technologies, we have developed interrelated solutions for yield enhancement and smart manufacturing. Focusing on realistic needs, novel solutions with data-driven UNISON framework for advanced quality control (AQC), advanced process control (APC), and advanced equipment control (AEC) have been developed on the basis of leading technologies such as big data analytics, artificial intelligence, Run-to-Run (R2R) control, virtual metrology (VM), fault detection and classification (FDC), and Prognostics and Health Management (PHM) based on big data of various factors involved in wafer fabrication.
In particular, we developed a UNISON framework to analyze the relationship between the intrinsic properties of wafers and the key performance index of the process variables of fabricated ICs for advanced quality control. The developed AQC can optimize the wafer specifications for the corresponding products for smart manufacturing as partial effort for enabling Industry 3.5. As chemical and mechanical processes are conducted on wafer substrates, the properties of the silicon wafers with different characteristics from various suppliers will affect the yield since critical dimensions are shrinking with less tolerance. Empirical studies have validated the proposed AQC solution that can suggest appropriate specifications of the raw materials to shorten the yield ramping time of a new product at advanced technology nodes.
Tool health monitoring and maintenance scheduling are crucial to empower smart manufacturing. Advanced Equipment Control, Prognostic and Health Management (PHM) are key solutions to enable Industry 4.0 via efficiently detecting the deviation of specific components and predicting potential faults to enhance tool health, reduce the maintenance cost, and avoid extreme failure events. A data-driven framework that integrates partial least squares and exponentially weighted moving-average was developed to deal with the multi-collinearity of equipment and process data for feature selection and model construction to monitor and predict tool health via analyzing the tool-status data collected from the sensors, and thus derive the optimal maintenance strategy for smart production. The results of an empirical study have validated the proposed approach for early detection of abnormal tool status, and to prolong the maintenance cycles for enhancing capacity utilization and productivity, and thus reduce the cost.
Overlays are designed for alignment between the present layer and previous layer for wafer fabrication. It is crucial to minimize overlay errors and control them within the tolerance to obtain high resolution and alignment accuracy for advanced technology nodes. We developed a novel dynamically adjusted proportional-integral (DAPI) run-to-run (R2R) controller to adapt equipment parameters to enhance the overlay control performance. Furthermore, we also developed a robust monitoring system for advanced control and compensation of the overlay errors based on E-insensitive support vector regression (SVR) while considering stochastic metrology delay (Fig.1). The results have shown the practical viability of this approach to effectively reduce the misalignment of the overlay errors through the self-tuning process, and to provide real-time decision support for process engineers.
Fig.1. Run-to-Run Control for overlay error compensation for advanced process control
These proposed solutions have been validated via empirical studies in leading semiconductor manufacturing companies. Indeed, the developed solutions have been implemented in real settings.
The paradigm of global manufacturing is shifting as leading nations return to manufacturing and propose the next phase of the industrial revolution such as Industry 4.0. Alternatively, I proposed Industry 3.5 as a hybrid strategy to address fundamental objectives for smart manufacturing for those who may not be able to migrate to Industry 4.0 directly. Furthermore, semiconductor manufacturing should become the keystone of the global manufacturing ecosystem and the leader to address sustainable growth and circular economy. Sponsored by the Ministry of Science & Technology, Taiwan, Artificial Intelligence for Intelligent Manufacturing Systems Research Center (AIMS) and my associates have integrated research and development efforts to empower Industry 3.5 smart manufacturing for other industries in Taiwan to humanize the ongoing industrial revolution and to share Taiwan Industry 3.5 smart manufacturing solutions to support the sustainable migration of emerging countries.
STAY CONNECTED. SUBSCRIBE TO OUR NEWSLETTER.
Add your information below to receive daily updates.