Cracking Mind’s Code
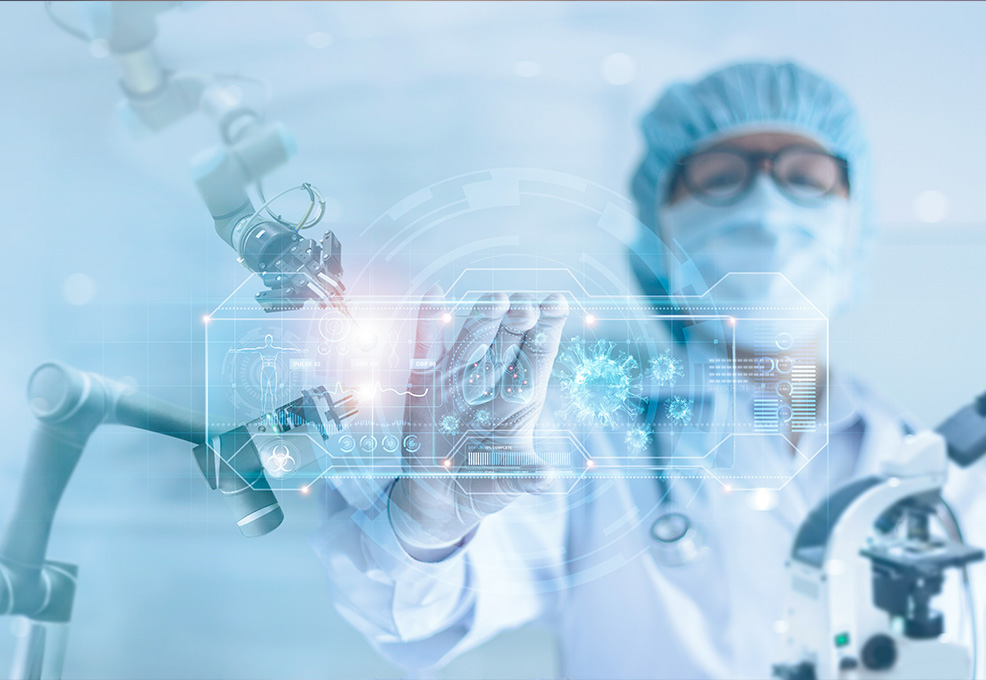
Author(s)
Ping-Yen LiuBiography
Dr. Ping-Yen Liu is a Professor of Cardiology, Director of the Institute of Clinical Medicine of National Cheng Kung University. He specializes in molecular biology and genetic research of arteriosclerosis diseases. He also focuses on deploying AI and smart medicine clinically, and the implementation of modern medical education.
Academy/University/Organization
National Cheng Kung UniversitySource
J Med Internet Res 2020; 22(10):e19878-
TAGS
-
Share this article
You are free to share this article under the Attribution 4.0 International license
- LIFE SCIENCES
- Text & Image
- November 23,2020
As COVID-19 became a pandemic, quarantine stations outside of emergency departments (EDs) at hospitals became necessary. It is a priority to simplify the workflow and decision-making process for physicians. We aim to demonstrate how the National Cheng Kung University Hospital artificial intelligence (AI) trilogy of diversion to a smart quarantine station, AI-assisted image interpretation, and a built-in clinical decision-making algorithm improves medical care and reduces quarantine processing times. This “AI trilogy” was applied to shorten the quarantine survey process and reduce processing time during the COVID-19 pandemic. The use of the AI trilogy facilitated the processing of suspected cases of COVID-19 with or without symptoms; also, travel, occupation, contact, and clustering histories were obtained with a tablet computer device. A separate AI-mode function that could quickly recognize pulmonary infiltrates on chest x-rays was merged into the smart clinical assisting system (SCAS), and this model was subsequently trained with COVID-19 pneumonia cases from the GitHub open source dataset. The SCAS algorithm was continuously adjusted based on updates to the Taiwan Centers for Disease Control public safety guidelines for faster clinical decision making. Our ex vivo study demonstrated the efficiency of disinfecting the tablet computer surface by wiping it twice with 75% alcohol sanitizer. The use of the AI application in the quarantine station significantly reduced the survey time. Thus, we concluded that this AI trilogy improved our medical care workflow by shortening the quarantine survey procedure and reducing the processing time, which is especially important during an emerging infectious disease epidemic.
Figure 1: The uncertainty in the air of COVID-19 quarantine station was above our imagination. With crowded staffs in a limited space, complexity of the decision strategy and judging whether the X-ray was reported as pneumonia or not made the whole story more high-pressured.
In December 2019, a local outbreak of pneumonia caused by a novel coronavirus, also called COVID-19, disrupted our lives. This coronavirus is considered to be extremely dangerous to human beings. Person-to-person transmission has been documented for COVID-19, and prevention of in-hospital outbreaks became a pertinent issue for crowded departments with highly-infectious regions, including emergency departments (ED), outpatient clinics and inpatient admission wards. With experience of SARS in 2003, Quarantine Stations (Q stations) outside of emergency departments at most hospital had developed a strategy to deal with the emerging disease. To prepare for the screen workload inside the Q station, most staff with medical licenses were required to support the working shift. Therefore, it is crucial to simplify the workflow and decision-making process for doctors from all subspecialist fields.
Furthermore, the dynamic situation changed every day with new CDC announcements, requiring a total of 53 immediate changing protocols. To fulfill the criteria and strategy for screening, and to shorten the process time in the COVID-19 Q station, National Cheng Kung University Hospital (NCKUH) designed an artificial intelligence (AI) system to solve these unmet clinical needs. The NCKUH AI team successfully developed a computer assisted technology device to accelerate medical decisions with their prior smart medicine research experience before the event. The goal was to reduce potentially dangerous SARS-CoV-2 exposure due to long duration times in the quarantine unit. In response to this increasing public health emergency, the NCKUH AI team developed a “smart Q station” outside of the NCKUH emergency room to facilitate the screening and survey procedure.
The Trilogy included: 1) diversion via the Q station; 2) a tablet computer for TOCC; and 3) an AI-assisted image interpretation with clinical strategy decision-making algorithms at the smart Q station. These steps efficiently facilitated the screening and survey process for those suspected cases at NCKUH.
For the expected high flow visit for this emerging infectious disease epidemic, NCKUH immediately decided to set up 8 temporary wartime quarantine tents for the Wuhan Pneumonia Quarantine Action. Simultaneously, a more permanent, structured house for long-term quarantine was initiated near the wartime tents. This effective diversion of patients from the ER to the quarantine tents relieved the crowded ER. On average, each medical staff member was estimated to have checked 35-50 quarantine patients per day.
Figure 2: The trilogy of scientific prevention in National Cheng Kung University Hospital: A. First, we used the precious space of the hospital to design a quarantine station that avoids the crowds between the outpatient building and in-patient emergency building; B. We also used smart tablet computers to fill in medical records by patients and collect patients' travel, occupational, cluster and contract histories, and imbedded decision trees into the program to help doctors and nurses; furthermore, with the cooperative work from AI engineers and medical imaging physicians, we also implant a wonderful AI COVID-19 image interpretation system; C. The establishment of these processes finally reduced the time for patients at the quarantine station from 120 minutes to 20 minutes.
Development of AI models for chest x-ray analysis
Development of AI models for chest x-ray analysis can ease the burden of medical staff and promote rapid triaging. In order to detect the precise location of pneumonia sites, we adopted a segmentation model with a class attention map (CAM). The pneumonia segmentation model was based on U-Net.
We thus built a quarantine station with a smart clinical assisting system (SCAS) to accelerate workflow. The SCAS is a clinical decision tree algorithm which integrates the structured format of TOCC history recordings, AI-assisted interpretation of chest X-rays, and the clinical recommended workflow. Suspected cases of COVID-19 at the quarantine station used the tablet computer for the SCAS questionnaires of TOCC history with adequate alcohol disinfection between the users. To avoid cross-infection between front-line medical staff and the patients, physicians and nurses all used independent computers.
We also retrospectively retrieved 4,000 chest x-rays from our picture archiving and communication system (PACS), and identified 682 chest posteroanterior (PA) x-rays with pneumonia and 692 of normal chest PA x-rays. Our AI mode for quickly recognizing pulmonary infiltrates on chest x-rays was merged into the SCAS, with sensitivity, specificity and accuracy of 94.1%, 95.1% and 94.6%, respectively using the training dataset. Furthermore, we used cases of COVID-19 pneumonia from one open source dataset in GitHub (https://github.com/ieee8023/covid-chestxray-dataset) including 59 PA and 11 AP chest x-rays to test the model. We achieved the detection rate of 93.2% in AP chest x-rays.
STAY CONNECTED. SUBSCRIBE TO OUR NEWSLETTER.
Add your information below to receive daily updates.