Forming the Solar System From Scratch With Hints From the Meteorites
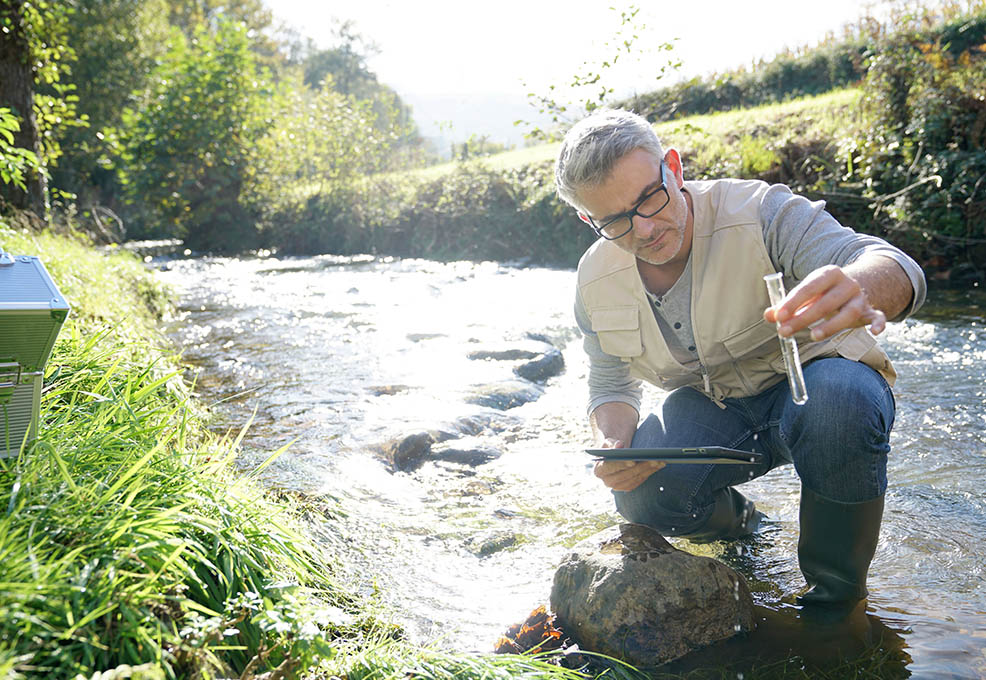
Author(s)
Chao-Hung LinBiography
Chao-Hung Lin is a professor of the Dept. of Geomatics, National Cheng Kung University, Taiwan. He was the department chair from 2017 to 2020. Professor Lin has served as chair of ISPRS Technical Commission V8, member of editorial board of Journal of Geomatics and Planning and International Journal of Computer Science and Artificial Intelligence.
Academy/University/Organization
National Cheng Kung UniversitySource
https://www.mdpi.com/2072-4292/12/12/1966-
TAGS
-
Share this article
You are free to share this article under the Attribution 4.0 International license
- NATURAL SCIENCES
- Text & Image
- October 22,2020
Nowadays, inland water pollution has become one of the global concerns that threatens public health and sustainable development. To know where and when the water is polluted and unsafe, our research team created a system, called WaterNet, for automatic water quality monitoring. With the combination of artificial neural network (ANN) and satellite technology, this system is able to monitor water quality from optical remote-sensing images. WaterNet addresses the estimation of chlorophyll-a (Chl-a) concentration, which is an important parameter linked to the change in water quality. High Chl-a concentration causes a decline in water quality. With the aid of the University of Philippines, a dataset containing 257 Chl-a samples was collected in Laguna Lake, the Philippines for the ANN model training. After the convergence of model training, the model shows good performance in the prediction of water quality. The interesting findings of this research are that the ANN-based model is obtained using a limited number of in-situ samples. This benefits researchers in terms of time-saving and labor cost reduction in training data collection. Besides, WaterNet can be a powerful tool for nationwide water quality monitoring.
Over the last decades, urbanization associated with the rapid growth of agriculture and industry has increased the nutrient loading in lakes, reservoirs, and rivers. The nutrient enrichment over a long period of time allows algae blooms to occur in water. This phenomenon can lead to massive fish deaths and a decline in water quality, posing serious risks to human health. Therefore, monitoring inland water quality plays an important role in water management.
Numerous methods are available to monitor water quality. The conventional method is to collect many in-situ samples to obtain the water quality information. This method is precise but time-consuming, labor-intensive, and expensive. In addition, this method is limited to the sampling locations, and so fails to obtain information for the entire water body. With the development of satellite technologies and the power of machine learning techniques, our team created WaterNet, which is an ANN-based system working on satellite images for automatic water quality monitoring. A total of 257 Chl-a in-situ samples were collected in Laguna Lake, the Philippines during several field campaigns by the research teams of National Cheng Kung University (NCKU) and the University of Philippines (UP).
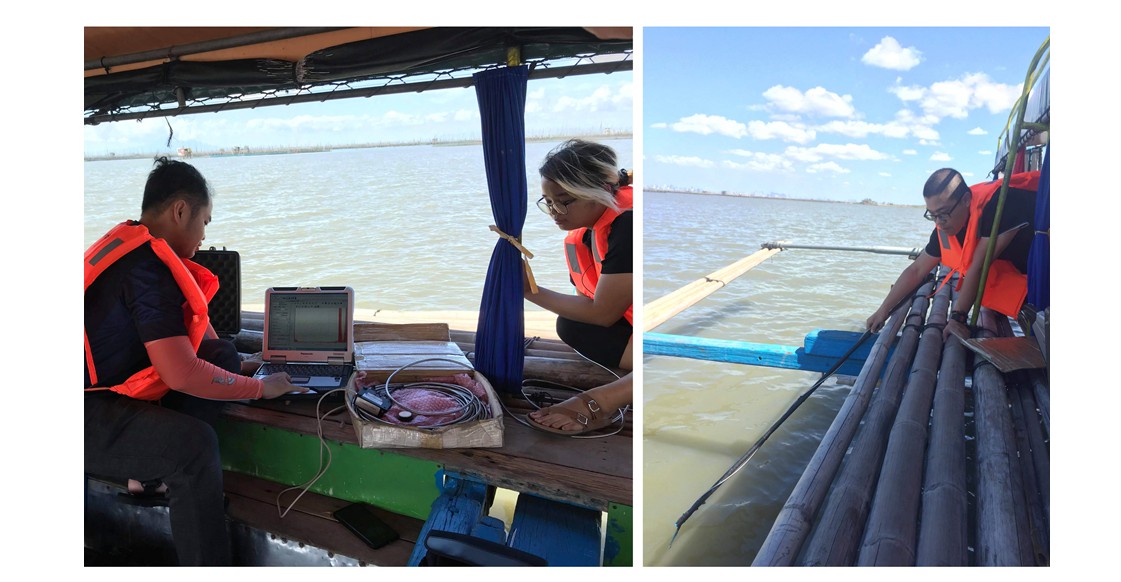
Figure 1. Water sample collection by the research teams of NCKU and UP on 30 April 2019 at the same time with the satellite flying across Laguna Lake.
Figure 2. Sentinel-3 satellite images of Laguna Lake, which is the largest lake located east of Metro Manila, the Philippines
To obtain a synoptic view of Chl-a concentration in the whole of Laguna Lake, our team utilized Sentinel-3 optical satellite images with a spatial resolution of 300 x 300 meters as the data source. After atmospheric correction, the spectral surface reflectance was input to WaterNet, and the Chl-a concentration was output from the water quality model. The ANN-based model comprises several convolution layers and two fully-connected layers. The input is an image patch of the size 7 (length) x 7 (width) x 16 (spectral) located at a sampling point. Both the spatial and spectral information of the sampling point are used in the Chl-a concentration estimation.
Figure 3. Network structure of the Chl-a concentration model, which consists of band expansion, feature extraction, and Chl-a concentration estimation.
Southeast Asian countries suffer economic losses of a million USD per year due to eutrophication. The prevention of water quality deterioration by monitoring Chl-a concentrations is important not only for the improvement of public health but also for the reduction of financial losses. WaterNet, which has been proven efficient in nationwide water quality monitoring, will be further applied to inland waters in Vietnam and Indonesia in accordance with the New Southbound Policy.
Figure 4. Spatial-temporal chlorophyll-a concentration maps in 2019. The white areas represent no information on satellite images due to cloud cover.
STAY CONNECTED. SUBSCRIBE TO OUR NEWSLETTER.
Add your information below to receive daily updates.