Windowed Fourier Transform Biosensing through Fano Resonance to Enhance Sensitivity
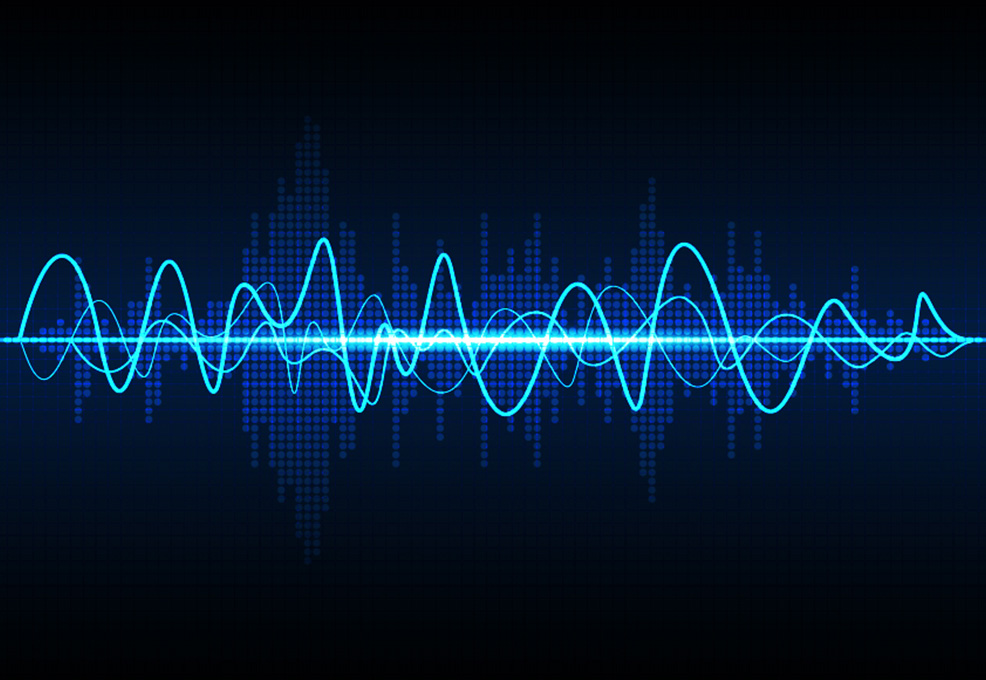
Author(s)
Yu-Ting TsaiBiography
Dr. Yu-Ting Tsai is an associate professor in the Bachelor’s Program in Precision System Design at Feng Chia University. His research is in the areas of mechanical and electrical integration, machine learning systems, optimization models, and sound processing technology.
Academy/University/Organization
Feng Chia University-
TAGS
-
Share this article
You are free to share this article under the Attribution 4.0 International license
- ENGINEERING & TECHNOLOGIES
- Text & Image
- August 20,2020
With the growth of Industry 4.0, a wide range of abnormal diagnosis and prediction technologies utilizing artificial intelligence (AI) have been extensively investigated in the field of mechanical diagnosis and prevention of system failures. The acoustic emissions machine learning (AEML) method is a data-driven health monitoring system that utilizes acoustic emission data, including vibration signals from mechanical equipment and acoustic images, to predict and prevent system failures. It utilizes deep learning models to analyze and combine input feature data that can then be used for decision-making problems of multiple objectives with AI learning methods. The system can be widely used in many situations, especially in monitoring machine status and product quality with high identification.
In Industry 4.0, there has been a keen interest in the development of technologies that combine AI technology with automation. The control and operation of a manual production line require engineers who have a preliminary subjective judgment of relevant manufacturing processes to determine the actual procedures used and the corresponding control settings. However, with such manual control setting methods, it is difficult to transform it into an automated production line such as the assembly process of multiple institutions. Therefore, it is expected that the emergence of a digital twin, or cyber-physical system, can use the domain knowledge in various fields to build their intelligent production systems, so that the AI can be realized by analyzing and developing the best applications via big data. Many industrial application techniques have utilized smart automation methods to achieve high-precision production models. At the quality control (QC) stage, the AEML method can use the frequency response methods to analyze the mechanical noise for estimating the product quality of production lines. As such, many manufacturers in Taiwan have recently begun to expect that this technology can be used to improve their product quality, and are seeking opportunities for industry-university cooperation in order to solve the scarcity problem of human resources.
The AEML method entails the use of acoustic emissions and automatic AI for industrial monitoring applications, making it closely related to the field of AI machine learning technology. The AEML technique aims to develop data-driven solutions based on the information from acoustics data, such as audio streams, acoustic images, the parameters of acoustic structures, acoustic features, and vibration signals from mechanical or audible equipment. The AEML method can recognize and analyze incoming data from the spectrum of the acoustical signal flow of each sensor, and undertake relevant classification tasks accordingly. Since acoustic sensors, such as microelectromechanical microphones (MEMS), are now widely available, applying the AEML method to the QC production line can be more economical. Compared with the traditional acoustic methods, the AEML method connected with the AI model can better identify and analyze the acoustic signal data of each sensor, and perform related quality inspection classification tasks accordingly. Some of the technologies employed in the AEML method, such as the convolutional neural networks (CNNs) and recurrent neural network (RNN) models, can be used to establish an integration model. After this model is trained, the effects and related useful information from within the input information can be used to analyze various aspects of a production line.
At the same time, the AEML method can also be applied to health monitoring devices to identify noise-related tasks. The abnormal vibration sounds generated by engine components are often indicative of their quality issues. In this case, the acoustic message characteristics and the AI classification model can also determine the product defect problem. Moreover, the AEML method can be used in the car motor health management systems. In such cases, the AEML method was applied to distinguish noise problems in car motors. The results indicated that the trained model ultimately achieved 93.2% prediction accuracy.
Many Internet of Things (IoT) and equipment suppliers and researchers have expressed interest in this application of the AEML method. In the future, it is also expected that it can be applied in bi-directional control processes in production systems. The control terminal for AEML methods and thread management systems can be integrated to establish an IoT control system to control the main thread and implement policies and operations.
Figure 1. Using acoustic emissions and automatic artificial intelligence for monitoring applications of industrial machine health
Figure 2. The general concept for processing acoustic emissions for use by the AI-based learning method
Figure 3. This example shows the application for automatic clustering of machine noise
STAY CONNECTED. SUBSCRIBE TO OUR NEWSLETTER.
Add your information below to receive daily updates.