Cone-beam computed tomography (CBCT) is a powerful visualization tool in digital dental treatment planning. According to the “as low as diagnostically acceptable” principle (ALADA), optimization of exposure to maintain diagnostic ability is currently an issue of concern for dental technology researchers. However, the anatomical structure complexity requires a compromise between radiation dosage and reconstructed image quality, which is addressed in dental implantology, maxillofacial/orthognathic surgery, orthodontics, and comprehensive maxillofacial-oral treatment and rehabilitation. Moreover, the productive image diagnostics is a key for treatment predictability, success, and patients’ greatest satisfaction. Motivated by these factors, the selective anatomy analytic iteration reconstruction algorithm (SA2IR) was developed to assure that the 3D data fusion visualization and planned treatment could be accomplished accurately and at an acceptable cost. To solve the problem of sparse low-dosed CBCT reconstruction (SLDCTR), SA2IR was proposed to enrich a number of input projections by interpolation, as a pseudo input, then to enhance their quality to meet the reconstructable requirement, which is mentioned as a prior reconstruction input optimization. This makes the reconstruction computation timing cheaper, while maintaining reasonable diagnostics quality. Currently, our proposed algorithm has been successfully demonstrated for oral and dentition anatomy structure, having significant generative noise reduction, faster implementation, structural similarity preservation and human compatible visualization, compared to the other gradient descent family and simultaneous iterative reconstruction family algorithms.
Since the 2010s, interest in Cone-beam computed tomography (CBCT) integration in modern computer-aided dentistry has gone viral in clinical practices and dental laboratory computer-aided design and manufacturing (CAD/CAM) infrastructures. The advanced radiology anatomical diagnostics empowers comprehensive data fusion visualization in the guided treatment planning, achieving higher predictability, greater patient acceptance, and high success rates while also being significantly safer. According to the computed tomography reconstruction principle, the computer synthesizes the raw input projections from various angles per scanning revolution/cycle, acquired by a radiology imaging flat panel detector. The reconstructed result is physically modified and converted into a standardized digital format in medical imaging and communication, called DICOM, as shown in Figure 1. Physically, the more intensity sensed by the detector, the more clinical information is given to the final reconstructed results, making it more diagnosable for clinicians. However, overexposure, exposure abuse and malfunctions are forbidden due to medical ethics and health concerns. According to the “as low as diagnostically acceptable” (ALADA) principle (Dr Bushberg, 2014), bio-physical radiation attenuation of human-beings must be optimized with fewer projections and lower dosage, while the informative imaging quality is maintained and should be diagnosable. This presents a new frontier for researchers to develop new technology approaches in clinical imaging and communication productivity enhancement in daily practices. Due to the importance of fusion anatomy analysis, the sparse low-dose CBCT reconstruction (SLDCTR) is a motivation for studies to be conducted for better public healthcare and benefits in digital dentistry. That also motivated us to focus on the field with selective anatomy analysis for proper reconstruction.
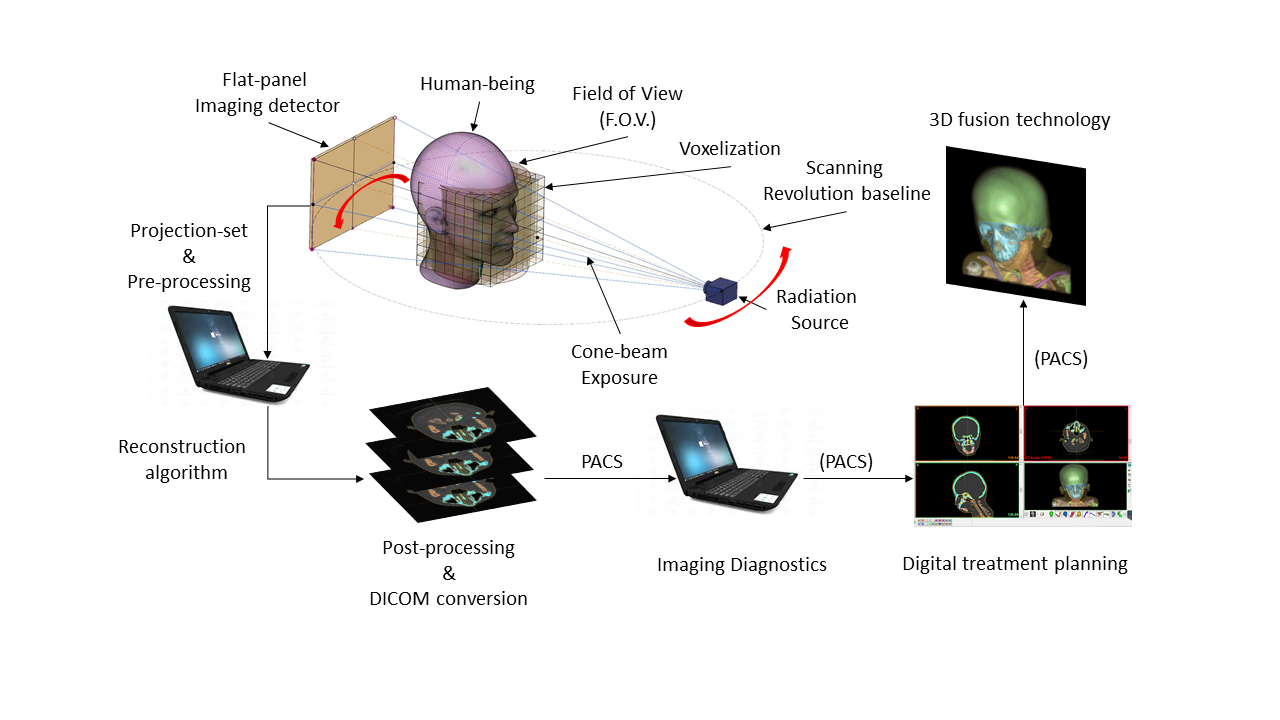
Figure 1. CBCT reconstruction and 3D fusion technology in digital dentistry (© 2011-2025, Lam Dao-Ngoc, DDS®).
Over a decade, many SLDCTR approaches have been proposed and used in CT technology, including sparse representation to compressed sensing (Candès et al., 2004), working with a limited number of input projections, lower than the optimum sampling theory of Nyquist-Shannon. With the recent flourishing of GPU-based computation, SLDCTR has achieved some cutting-edged applications. However, in order to produce a high-grade clinical imaging reference, not only the computational complexity and expensive costs, but also the ambiguous tissue behaviors make the relevant studies exhausted and “knapsack-like” tuned,. Medically, due to the lack of productivity and compromisable collaboration with expert clinicians and pathological understanding, technology has not supported making clinical decisions safer or much more efficient. Technically, the limitation of in-vivo experiments in bio-physical tissue x-ray attenuation (BPA) makes the parameter tuning simulated on the physical equivalent BPA phantoms only. The most recent studies are approached by computation optimization techniques, or statistical modeling of the operation system of CBCT and exposure behavior; however, that does not fully solve the ambiguity of the reconstructed anatomical features.
We proposed a new algorithm for SLDCTR, which solves the under-sampling issue and improves the projection quality. Firstly, regarding the specifications of the CBCT design, we interpolate and generate additional projections with proper overlapping conditions, then insert them into the raw input projection-set (IPS) to make its sampling effectively reconstructable. Secondly, the newly generated set is called the “pseudo projection-set” (pIPS), in which each projection quality is improved in three steps: (a) the 1st step is to estimate the updated projection by its prior projection, as an “estimation operator,” (b) the 2nd step is to filter it by convoluting itself to reduce minor backgrounded defects, as a “filter operator,” and (c) the 3rd step is to verify the updated projection by its posterior projection, as a “verification operator.” This three-step image quality enhancement is called “trio-subset enhancement” (shown in Figure 2). The updated projection improves the spatial anatomical similarity before reconstruction. Finally, the updated projection-set is reconstructed by the self-tuned simultaneously iterative reconstruction technique. Computationally, the arithmetic processing makes reconstruction implementation much faster with GPU-support, in comparison to some other common algorithms (shown in Table 1). This is why the proposed algorithm is named the “Selective Anatomical Analysis Iterative Reconstruction” algorithm (SA2IR) (Dao-Ngoc, L. and Du, Y.-C., 2019).
Figure 2. Illustrations of the projection set and the trio subset of SA2IR.
The main outcomes of our proposed algorithm are presented as follows:
1. Enrichment of the input projection-set (IPS): The number of input projection-sets is increased by interpolation and insertion of the new projections from the original set at the common angles of the IPS and the desired full-set. The pIPS is quantitatively and qualitatively adapted to the generalized CT reconstruct-ability, which is normally from 300 projections (standard resolution mode) to 600 projections (high-resolution mode) per scanning revolution (shown in Figure 3).
Figure 3. The IPS enrichment of SA2IR, implemented on realistic patient data
(entropy of original (blue), IPS (orange), and pIPS (purple))
2. Enhancement of the pseudo input projection-set (pIPS): The quality of pIPS is enhanced by a sequence of three successive projections, which is optimal for the successive projection continuity. The proposed trio-subset enhancement is pixel-wise improved with the informative anatomical features, which makes the following reconstruction process implementation smoother and faster.
3. Modified Iterative Reconstruction: the self-tuned simultaneous iterative reconstruction algorithm is proposed. The computation speed is proved to be significantly faster than the gradient descent algorithms and the simultaneous algebraic iterative reconstruction algorithms. Furthermore, diagnostic ability is maintained, due to the significantly generative noise reduction, structural similarity enhancement, and human vision contrast compatibility.
Table 1. Computation speed of the proposed SA2IR compared to the others
4. Compliant with ALADA requirements and beneficial to dental treatment practices: SA2IR is proved to be effective and highly applicable in dental SLDCTR applications, with realistic patient head data. The demonstrations are implemented in different anatomical regions of interest, such as the upper jaw region, lower arch region, middle facial region, and temporomandibular joint region, as shown in Figure 4. Obviously superior in image quality and diagnosability compared with all the other results, the results of SIRT have the shape and boundary of bones and teeth much more blurred, thicker and with lower contrast. The image details of bone and teeth in the results of SART and ASD-POCS are slightly better in terms of visibility due to the high contrast, but there are many more streaking artifacts than in the SA2IRT results. Furthermore, the general morphology of bones, teeth and transitional junctions is maintained as diagnosable in the results of SA2IRT due to the least amount of salt noise, streaking artifacts and much more visual compatibility in comparison with all the other results. Especially in the mid-facial and temporomandibular regions, the morphological similarity of all the anatomical tissues is comparable from SA2IRT to SART and ASD-POCS. Moreover, in the sampling (sparse and low-dose) conditions which shown in Fig. 4, the narrow min-max discrepancy of contrast is non-beneficial for both ASD-POCS and SART without any compromises with post-processing, while it would be enhanced with the SA2IRT pre-processing steps to reduce the overlapping inadequacies. In general, SA2IRT is more ALADA adaptive, has reasonable diagnostic ability, and can be implemented faster, while the patient has less exposure time, number of projections, and dosage, benchmarked to the other mentioned results.
Figure 4. The reconstructed results of other algorithms compared to SA2IR, with respect to the different oral and dental anatomical regions.
According to our developed algorithm’s results, the selective anatomy analysis approach is well-designed in raw data pre-processing of SA2IR reconstruction workflow for SLDCTR problems. The motivation of ALADA adaptiveness and planned treatment effectiveness will make our future studies on the selective anatomy analysis application more practical. The thinking of mapping the bio-physical attenuation behavior of oral and dentition tissue structures, termed in statistical morphology modeling, will inspire us to verify and improve our proposed algorithm applicability in real-life digitalized dentistry.