Sensors-The Senses of Smart Machinery
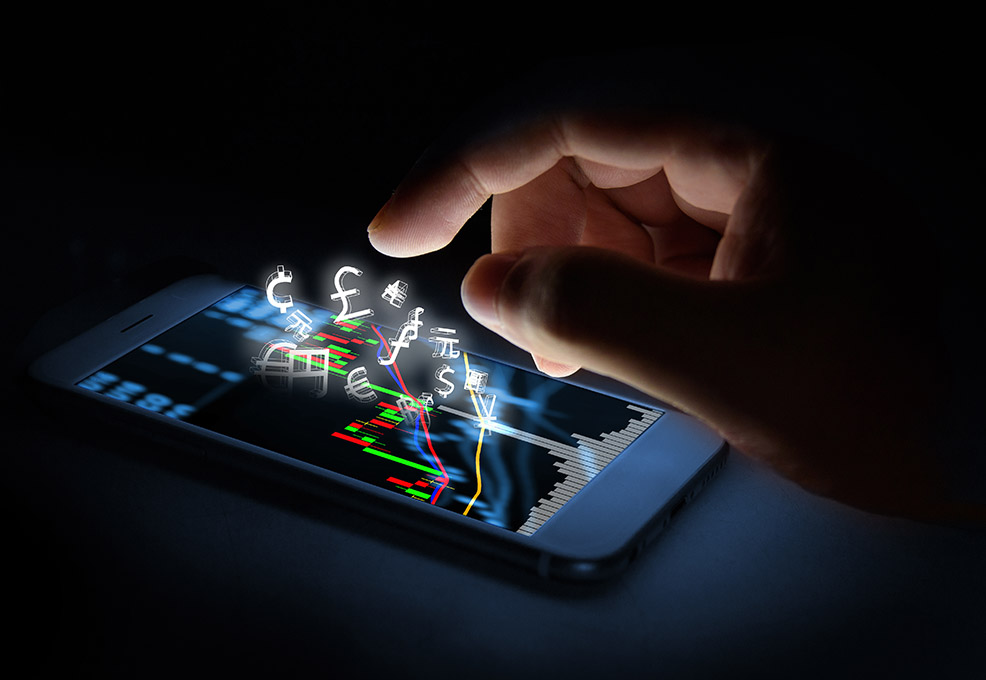
Author(s)
Chung-Chi Chen & Hsin-Hsi ChenBiography
Chung-Chi Chen is a PhD candidate in the Department of Computer Science and Information Engineering at National Taiwan University. He received his M.S. degree in Quantitative Finance from National Tsing Hua University, Taiwan. His research focuses on opinion mining and sentiment analysis in financial social media.
Prof. Hsin-Hsi Chen is a distinguished professor in the Department of Computer Science and Information Engineering, National Taiwan University. He serves as director of MOST Joint Research Center for AI Technology and All Vista Healthcare. His research interests are natural language processing, information retrieval and extraction, and web mining.Academy/University/Organization
National Taiwan UniversitySource
https://ieeexplore.ieee.org/abstract/document/8609586
https://doi.org/10.1145/3308558.3314122-
TAGS
-
Share this article
You are free to share this article under the Attribution 4.0 International license
- ENGINEERING & TECHNOLOGIES
- Text & Image
- June 18,2019
Social trading platforms provide a forum for investors to share their trading ideas. They are one of the disruptive innovations in the recent FinTech (Financial Technology) trend. Sophisticated individual investors on the social trading platforms provide ponderable opinions for investment, and attract many followers. They corrode the value of industrial professionals. Someday soon, these investors will become the celebrities of the amateur investors just like the YouTubers in the entertainment industry.
Differing from traditional social network platforms on which most users share lifelogs such as travel or meals, social trading platforms provide a forum for individual investors to share their trading ideas, strategies, and opinions. Such a special kind of social network platform is considered as the next big hit in the FinTech trend. Like the YouTubers who have eroded the value of the entertainment industry, the sophisticated investors on the social trading platforms have been corroding the value of the wealth management industry. Bloomberg and Reuters provide market information such as real-time market data, news, and research reports. The information in the reports of analysts has been well sorted out into a structured form. For example, price target (PT), the forecast price level that investors believe the price of certain financial instruments will achieve, is highlighted in the reports. However, the opinions of the crowd still remain at the sentiment level. Along this line, we attempt to sort out the fine-grained opinions of the crowd via numerical understanding techniques.
Numerals provide rich and crucial information in documents in many domains. For example, in clinical records, one important piece of information is dosage, expressed by numerals; numerals provide ingredient proportions in recipes; in financial narrative, numerals represent up to 17 meanings in the taxonomy of our previous work. These examples show that fine-grained analysis of numerals is worthwhile. In our demonstration, called CrowdPT, we focus on summarizing the crowd opinions into one numeral, price target, to show not only the bullish/bearish sentiment of the crowd, but also the exact price level that will be expected.
Figure 1 illustrates the flowchart of price target extraction. Firstly, we monitor the latest tweets published on Twitter. Only the tweets mentioning the cashtags of the constituent stocks of the Dow Jones Index will be crawled. Secondly, the price target filter is performed to sort out the price target for each cashtag. Then, a classifier based on a convolutional neural network (CNN) is adopted to extract the price target. Finally, we collect the close price from Yahoo Finance and render the price chart.
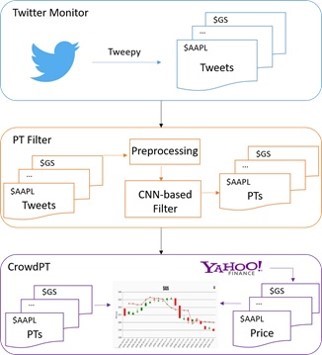
Figure 1: Flowchart of CrowdPT
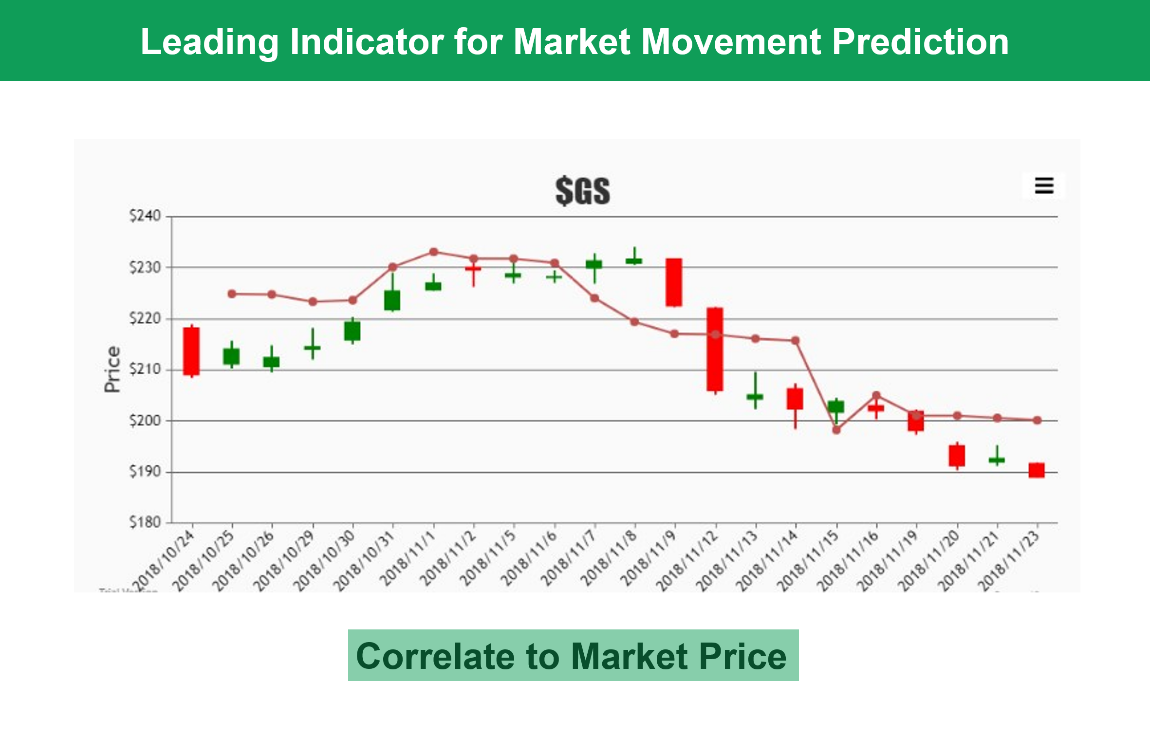
Figure 2: Case study of the crowd’s PTs
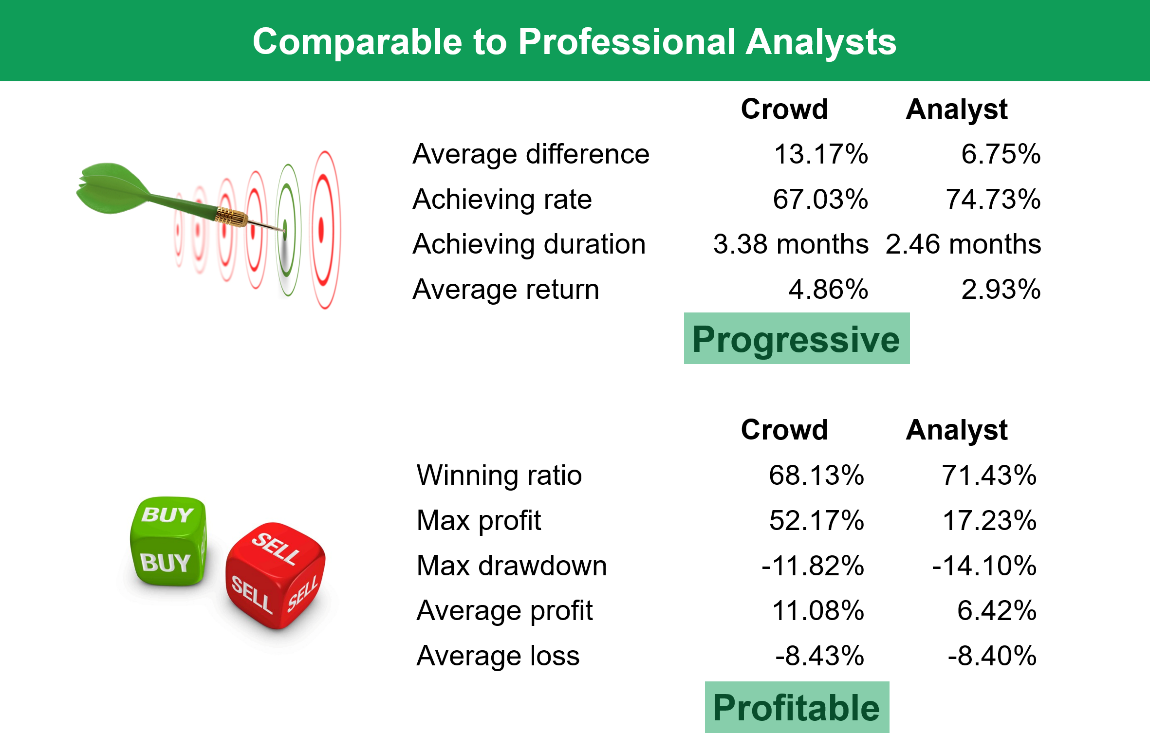
Figure 3: Comparison of crowd’s PTs and analysts’ PTs.
STAY CONNECTED. SUBSCRIBE TO OUR NEWSLETTER.
Add your information below to receive daily updates.