Intelligent Control of Grid-Connected Microgrid with Virtual Inertia
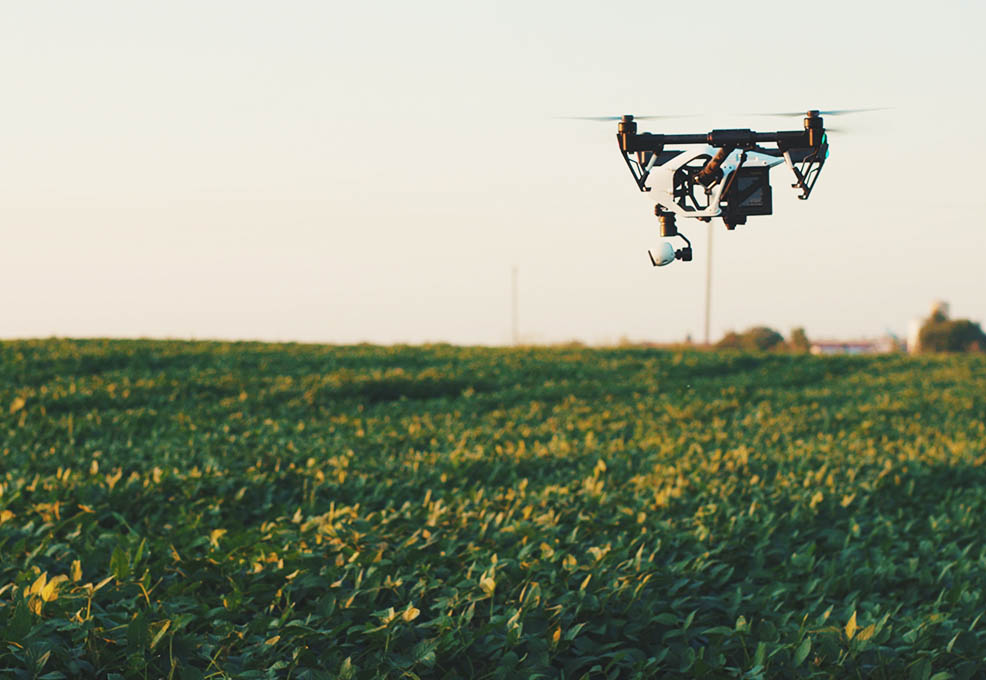
Author(s)
Ming-Der YangBiography
Professor Yang serves as a chair of the Department of Civil Engineering and the Unmanned Vehicle Research Center in National Chung Hsing University. Recently his research has focused on the applications of UAV image processing and deep learning technology in 3D model construction, disaster monitoring, agricultural production and loss assessment. He has twice won Breakthrough Awards for “Real-time identification of crop losses using UAV imagery” at the 2019 Future Tech Expo and for “Air/ground cooperation for optimal rice harvesting model” at the 2020 FUTEX.
Academy/University/Organization
National Chung Hsing UniversitySource
https://doi.org/10.3390/rs12040633-
TAGS
-
Share this article
You are free to share this article under the Attribution 4.0 International license
- ENGINEERING & TECHNOLOGIES
- Text & Image
- October 22,2020
Unmanned aerial vehicles (UAVs) have been rapidly developed in recent years, and have the advantages of low cost and high maneuverability, which has dramatically increased their popularity and applicability. However, the large number of images obtained by unmanned aerial vehicles also need innovative image processing technologies for information extraction. This research introduces employing deep learning methods to efficiently process a large number of UAV images and provide timely and accurate quantitative data for large-scale agricultural disaster investigations, which can further serve as the basis for agricultural disaster relief and agricultural insurance. In this study, two neural network architectures of image semantic segmentation models (FCN-AlexNet and SegNet) were established, and their accuracy and computational efficiency were compared. These two image semantic segmentation models were applied to UAV images to estimate the proportion of large-scale rice lodging. A set of 2017 UAV images was used to train and test the rice lodging identification models, which were verified by another set of UAV images obtained in 2019. The FCN-AlexNet rice lodging identification model had better performance with an F1-score of 0.82 and accuracy of 0.93. Compared with traditional classifiers such as maximum likelihood, the proposed semantic segmentation network models have higher efficiency and a lower error rate. In the future, the rice lodging identification models can be deployed on multiple computers for parallel computing to cope with large-scale agricultural disaster investigations.
Due to the development of advanced remote sensing techniques in recent decades, unmanned aerial vehicles (UAVs) have been rapidly developed in recent years. UAVs have the advantages of low cost and high maneuverability, which has dramatically increased their popularity and applicability for various real-world problems such as disaster impact assessment and land cover change. Nowadays, agricultural damage assessment heavily relies on manual evaluation, which is time-consuming, labor-intensive, subjective, and problematic in terms of its poor efficiency and objectivity. UAV imagery with fine temporal and spatial resolutions provides full coverage of crop fields with economic benefits, and so could be a prospective approach for recording the damaged scenes. However, the large number of images obtained by unmanned aerial vehicles also needs innovative image processing technologies for information extraction.
This research introduces the employment of deep learning methods to efficiently process a large number of UAV images and to provide timely and accurate quantitative data for large-scale agricultural disaster investigations, which can further serve as the basis for agricultural disaster relief and agricultural insurance. Two neural network architectures of image semantic segmentation models (FCN-AlexNet and SegNet) were employed, and their accuracy and computational efficiency were compared. FCN-AlexNet, a customized model based on the AlexNet architecture, has the advantages of a deeper network, and replaces the fully connected layers of AlexNet with a 1x1 convolution layer and a 63x63 upsampling layer for pixel-wise end-to-end semantic segmentation. Additionally, FCN can accept input images of any size, and retains the pixel spatial information in the original input image, which can classify each pixel on the feature map. SegNet has an asymmetric network architecture structure, including an encoder consisting of convolution layers and pooling layers, a decoder consisting of upsampling layers and convolution layers, and then a softmax layer. The encoder structure is identical to the 13 convolutional layers in the VGG16 network without the fully connected layers. Following the encoder, the decoder has a structure symmetric to that of the encoder, but employs upsampling layers instead of transpose convolution. These two image semantic segmentation models were applied to UAV images to estimate the proportion of large-scale rice lodging. A set of 2017 UAV images was used to train and test the rice lodging identification models, which were verified by another set of UAV images obtained in 2019. Both the FCN-AlexNet and the AlexNet models performed well in the training and validation. The FCN-AlexNet rice lodging identification model resulted in an F1-score of 0.83 and an accuracy of 0.94, whereas the AlexNet rice lodging identification model resulted in an F1-score of 0.79 and an accuracy of 0.92.
Compared with traditional classifiers such as maximum likelihood, the proposed semantic segmentation network models have higher efficiency (an increase in speed of about 10 to 15 times) and a lower error rate. The rice lodging map provides a timely and accurate quantitative reference for loss evaluation and disaster relief. In the future, the rice lodging identification models can be deployed on multiple computers for parallel computing to cope with large-scale (up to a hundred thousand hectares) agricultural disaster investigations. Edge computing techniques with hierarchical image processing in UAV-equipped microcomputers can also be applied to the deep-learning model to provide a real-time agricultural disaster survey.
STAY CONNECTED. SUBSCRIBE TO OUR NEWSLETTER.
Add your information below to receive daily updates.