From Hot-Jupiter Formation to Star Formation
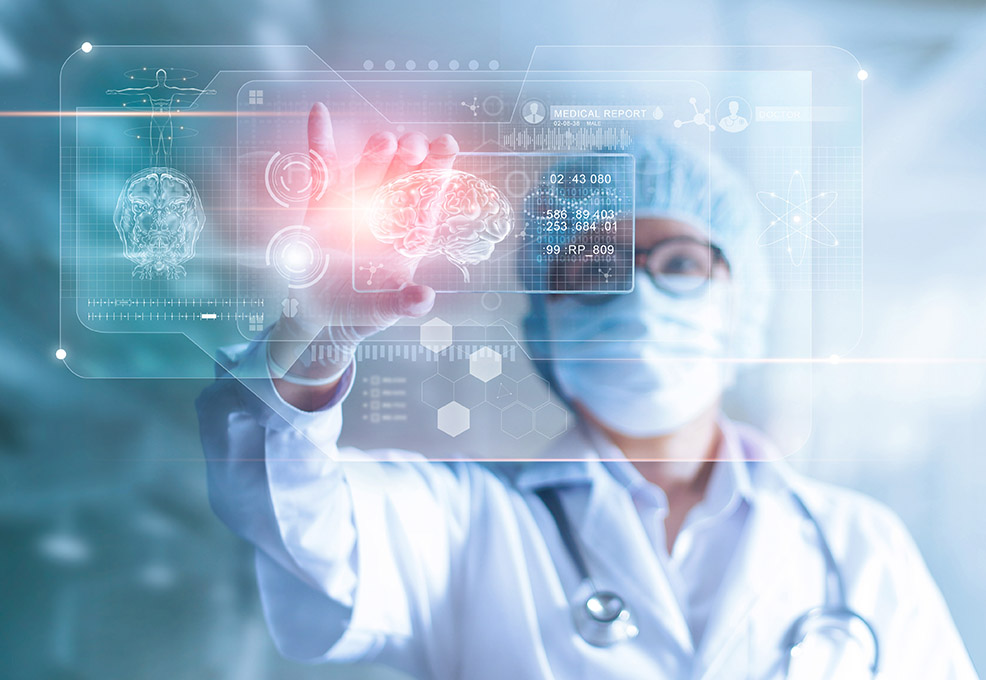
Author(s)
Chia-Yen LeeBiography
Prof. Lee is currently the director and associate professor in the Institute of Information and Systems at National Cheng Kung University (NCKU), Tainan, Taiwan. His research interests include data science, intelligent manufacturing systems, productivity analysis, and multi-criteria decision analysis.
Academy/University/Organization
National Cheng Kung UniversitySource
Lee, Chia-Yen, and Tsung-Lun Tsai, 2019. Data Science Framework for Variable Selection, Metrology Prediction, and Process Control in TFT-LCD Manufacturing. Robotics and Computer-Integrated Manufacturing, 55, 76-87.
Lee, Chia-Yen, and Chia-Lung Liang, 2018. Manufacturer's Printing Forecast, Reprinting Decision, and Contract Design in the Educational Publishing Industry. Computers & Industrial Engineering, 125, 678-687.
-
TAGS
-
Share this article
You are free to share this article under the Attribution 4.0 International license
- NATURAL SCIENCES
- Text & Image
- June 18,2019
This study describes the data science techniques applied in the manufacturing systems. These techniques can be applied in a variety of manufacturing fields, such as capacity planning, inventory management, price and demand forecasting, quality control, equipment maintenance, etc. This study introduces two cases in the literature: inventory control and metrology prediction, which may bring new insights and inspire our shop-floor level in the manufacturing system. The first empirical study focused on the inventory control of the educational publisher in the supply chain. The second empirical study focused on the process diagnosis and metrology prediction of the high-tech manufacturer. The results showed that data science techniques can improve the accuracy of demand forecasting, optimize the configuration of the manufacturing process and reduce the inventory level to enhance the business core competence.
Data science and machine learning are cutting-edge techniques applied in our real world. In the manufacturing system, there are several issues that urgently need to be solved such as capacity planning, inventory management, price and demand forecasting, quality control, equipment maintenance, etc., which significantly affect the profit or cost for business sustainability. This study introduces two cases in the literature which may bring new insights and inspire our shop-floor level in the manufacturing system.
The first case study focused on inventory control in the supply chain (Lee and Liang, 2018). An empirical study of the educational publishing industry was conducted and we found that the educational publisher generally built a huge amount of inventory for make-to-stock production and suffered the long-whip effect due to intransparent information in the supply chain. In fact, the manufacturer usually over-produces to satisfy the retailers’ demand; however, frequent revisions cause obsolescence and inventory scrap problems. In this case, the data science and optimization method can be used to develop a two-stage analysis, as shown in Figure 1. Figure 1 describes the problem definition, data preparation and preprocessing, demand forecast module, and capacity decision module. In the data preparation stage, sales data and population are collected and then data quality is improved by removing outliers and smoothing the noise. In the first stage, we predict the demand for a variety of print products by using a neural network, autoregressive integrated moving average (ARIMA), and a regression model. We integrated the predicted sales quantities generated from three types of forecast models, and investigated the pros and cons of the three models to develop a mechanism for improving the accuracy of the demand forecast by dynamic weighting adjustment. In the second stage, the capacity decision model was developed to control the production and optimize a tradeoff between capacity surplus and capacity shortage by using the linear programming technique to build a minimax regret model and stochastic programming model. In particular, the costs of unsold goods (i.e., capacity surplus) and stock-out (i.e., capacity shortage) are investigated by top management and updated periodically. The results of this empirical study showed that the proposed two-stage analysis can effectively improve forecast accuracy by 3.7% and further reduce costs by 8.3%.
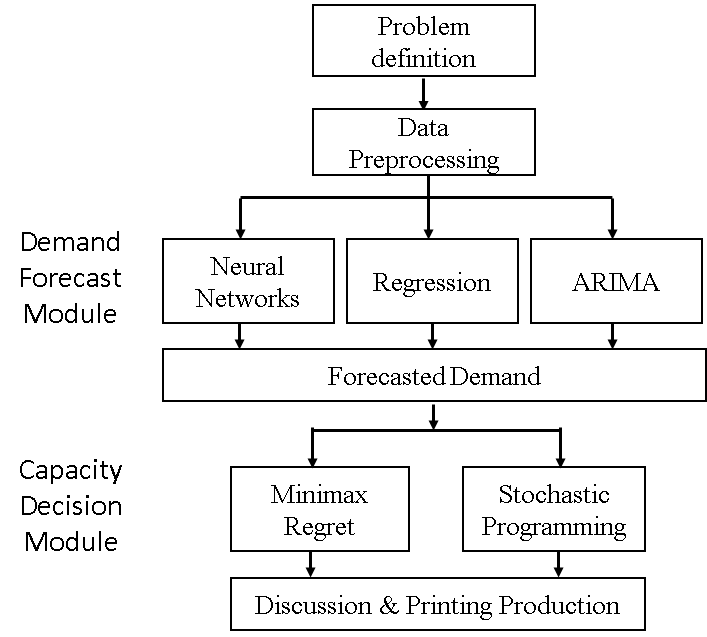
Figure 1 Printing forecast and production optimization (revised from Lee and Liang, 2018)
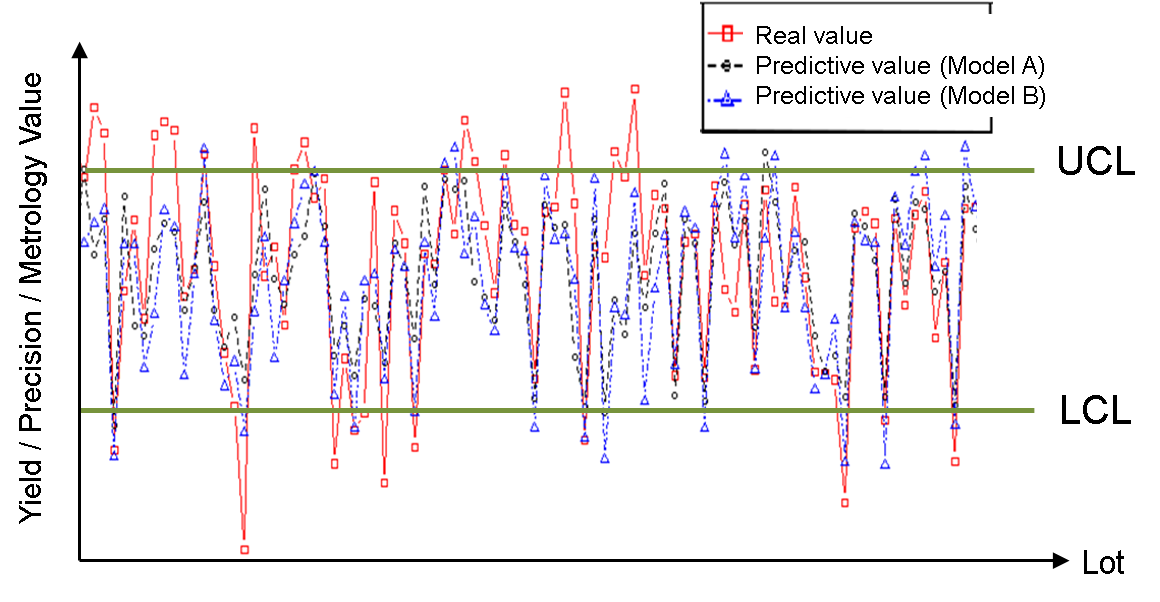
Figure 2 Metrology prediction
STAY CONNECTED. SUBSCRIBE TO OUR NEWSLETTER.
Add your information below to receive daily updates.