Indoor Localization of Mobile Vehicles for Intelligent Manufacturing Task Planning Based on Game Machines
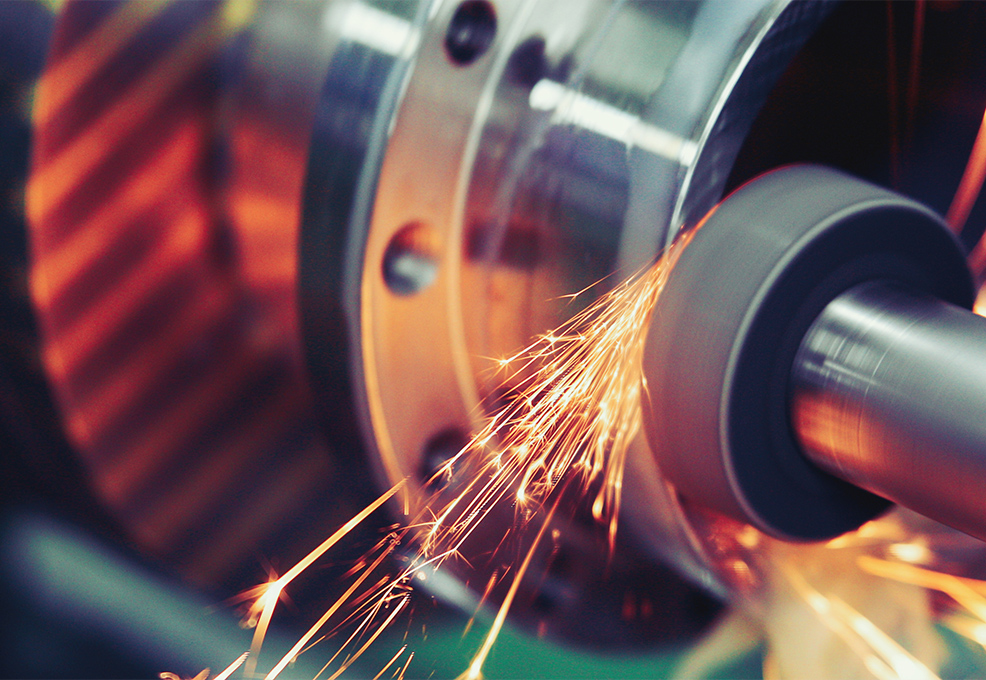
Author(s)
Her-Terng YauBiography
Professor Her-Terng Yau obtained his M.S. and Ph.D. degrees from National Cheng Kung University in 1996 and 2000, respectively. In 2009, he was invited to be a Professor in the Department of Electrical Engineering of National Chin-Yi University of Technology. At present, he is a distinguished professor, IET Fellow and technical editor of IEEE/ASME Transactions on Mechatronics.
Academy/University/Organization
National Cheng Kung UniversitySource
https://ieeexplore.ieee.org/document/8715781-
TAGS
-
Share this article
You are free to share this article under the Attribution 4.0 International license
- ENGINEERING & TECHNOLOGIES
- Text & Image
- August 20,2020
The intelligent detection and prediction technique for machine tool wear aims to transfer measurement signals into images by utilizing dynamic error attractors of fractional-order chaotic systems. Artificial intelligence and deep learning methods are carried out afterwards to distinguish and predict different conditions of tool wear. The technique not only surpasses the existing technology of the world-renowned manufacturing firms, but is also applied in domestic firms. The technique is a breakthrough technology for the discrimination and prediction of tool wear for the domestic machine tool industry in the future.
Major global machine tool manufacturers have published technical publications and practices on machine tool wear. By utilizing the chaotic attractor in the dynamic error mapping conversion technique that the research team applied, the lack of accuracy and immediacy of the existing technology due to the use of the traditional frequency domain can be further improved. The technique can transfer vibration signals into images, and with artificial intelligent (AI) methods, the images can be recognized to discriminate and predict the tool wear condition. The technique can be described in two aspects as follows.
First, as in the feature extraction of tool wear condition, dynamic errors of fractional-order chaotic systems are being used to detect the minor signal variations in different levels of tool wear condition. The measured signals transferred to images by chaotic map attractor noticeably show the variations in different wear patterns. The second aspect of the technique is the smart diagnosis and prediction. To identify the manufacturing and tool wear status, the transferred images are applied to deep learning convolutional neural networks (CNN). The tool life prediction is then carried out by the barycentric coordinate system figure which is transferred and mapped through chaotic dynamic error by the Grey System Theory. With the visualization of the quantity index, the manufacturing and tool wear monitoring technique is seen as a bold step away from the traditional method, and is also an important step forward within the smart machinery industry. Besides, the validation testing has already been done in a MOST project called “The Smart Functional Technique Development in Domestic Lathe Machine Tool Spindle.” With the collaboration of domestic machine tool manufacturers and spindle factories, it has successfully applied the analysis and prediction to the tool wear condition, which also provides a predictive solution for bearing systems in the Taiwan machine tools industry in developing the smart machinery policy.
In addition, there are several scientific breakthroughs when developing this technology. In terms of academic breakthrough, the research team led by Professor Yau is the first to propose the research of Feature Extraction in Electrical and Mechanical System Fault with Fractional-order Chaotic Dynamic Error Conversion, according to the IEEE Xplore and Thomson Reuters ISI Web of Science database. Correlational studies have already been published in several international journals include the IEEE ACCESS 2019, IEEE Transactions on Smart Grid 2017, IEEE Transactions on Industrial Electronics 2016, IEEE Trans. on Mechatronics 2013, IEEE Journal on Emerging and Selected Topics in Circuits and Systems 2013. On the other hand, as regards industrial innovation, the technique was patented in July 2019. Products adopting this technique will be launched at the end of 2020. It is expected to assist factories in enhancing their smart technology and competitiveness. Relevant research has also been published in international journals.
The application of Intelligent Fractional-order Chaotic System for Dynamic Error Conversion Feature Extract has been applied to the processing states monitoring and prediction in Intelligent Machinery and Intelligent Manufacturing. It can enhance the international competitiveness for domestic machine tool factories and traditional process industries.
STAY CONNECTED. SUBSCRIBE TO OUR NEWSLETTER.
Add your information below to receive daily updates.